Artificial intelligence (AI) is advancing at a breakneck pace, raising questions about its impact on various professions. One of the most intriguing debates centers around whether AI will eventually replace machine learning engineers. These professionals are the very architects of AI, so the idea of their own creations taking over their jobs is both fascinating and a bit unsettling.
As AI systems become more sophisticated, they can automate many tasks traditionally handled by human engineers. However, the relationship between AI and machine learning engineers is more complex than a simple replacement scenario. It involves collaboration, innovation, and a deep understanding of both technology and human creativity. This article delves into the evolving roles of AI and machine learning engineers, exploring whether AI will complement or compete with human expertise in this dynamic field.
Understanding AI and Machine Learning
AI and machine learning are closely related but distinct fields. Both play critical roles in modern technology and innovation.
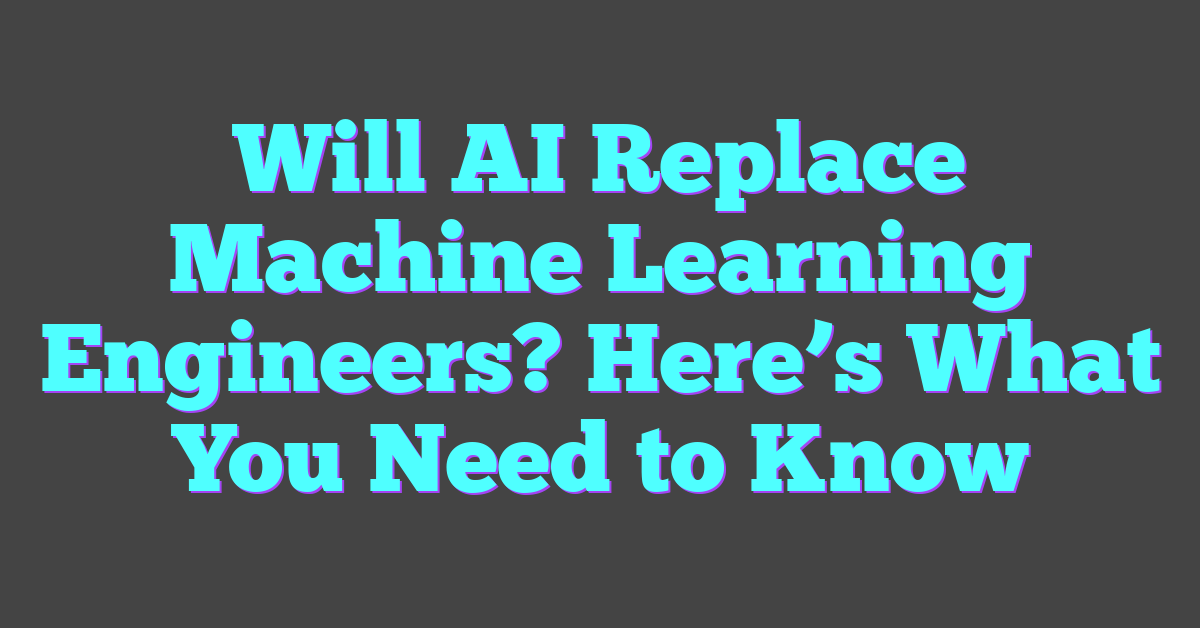
Defining AI
AI, or artificial intelligence, refers to the simulation of human intelligence in machines. These machines perform tasks that typically require human intelligence, such as problem-solving, learning, and decision-making. AI systems range from narrow AI, which is specialized for specific tasks like speech recognition, to general AI that can perform any intellectual task a human can.
Understanding Machine Learning
Machine learning, a subset of AI, involves training algorithms to learn from and make predictions based on data. It’s characterized by its reliance on large datasets and statistical analysis to improve performance over time. Notable examples include recommendation systems and image recognition. Machine learning models continuously evolve, enhancing their precision through iterative learning processes.
The Role of Machine Learning Engineers
Machine learning engineers are pivotal in developing AI systems, bridging the gap between data science and software engineering. They design and implement machine learning models to solve complex problems.
Key Responsibilities
Machine learning engineers handle diverse tasks:
- Developing Algorithms: They create and optimize algorithms for data processing and predictive analysis.
- Data Management: They prepare, clean, and organize data for training models, ensuring high-quality datasets.
- Model Training: They train models using large datasets, often employing deep learning techniques.
- Performance Evaluation: They evaluate model performance with metrics, refining algorithms to improve accuracy.
- Deployment and Maintenance: They deploy models into production environments and continuously monitor their performance, adjusting as needed.
Importance in Tech Industry
Machine learning engineers hold critical roles in the tech industry. Companies rely on their expertise to:
- Innovate Products: Engineers develop intelligent features in applications like recommendation systems and autonomous vehicles.
- Enhance Decision-Making: Their models provide data-driven insights, assisting businesses in strategic decisions.
- Automate Processes: They create automated solutions that streamline operations, increasing efficiency.
- Drive Research: Engineers contribute to cutting-edge research in AI, propelling technological advancements.
- Ensure Scalability: They build scalable systems capable of handling large volumes of data, crucial for big data applications.
Machine learning engineers’ contributions are foundational in advancing AI technologies, ensuring robust, efficient, and innovative solutions across various sectors.
AI Advancements and Automation
AI advancements are transforming the tech landscape, impacting various sectors and automation processes.
AI in Automation
Automation leverages AI to streamline tasks traditionally managed by humans. AI in automation enhances efficiency by enabling systems to perform repetitive tasks with precision. Manufacturing uses robots to assemble products. Customer service employs chatbots to handle inquiries. These applications illustrate AI’s capacity to minimize human error and increase productivity.
AI automates complex data processes, extracting insights from vast datasets. Financial services utilize AI for fraud detection. Healthcare integrates AI to analyze medical records. These integrations free up human experts to focus on more strategic tasks, driving innovation and improving service quality.
Recent Breakthroughs in AI
Recent breakthroughs in AI underscore its rapidly evolving nature. Major advancements include deep learning and neural networks. AlphaGo’s victory over a world champion Go player demonstrated AI’s advanced problem-solving abilities. GPT-4, a language model, showcases AI’s potential in natural language processing.
Reinforcement learning has improved robotic controls, with applications in autonomous vehicles and adaptive learning systems. AI’s image recognition capabilities have transformed medical diagnostics by identifying anomalies in X-rays and MRIs with high accuracy.
Continued research and development propel AI towards new frontiers, enhancing its capabilities and expanding its applications across industries. From predictive analytics to autonomous systems, AI’s progress signifies its integral role in shaping the future of technology.
Impact of AI on Machine Learning Jobs
Artificial intelligence (AI) is rapidly evolving, significantly impacting various roles, including those of machine learning engineers. This section details these changes and explores the implications for the industry.
Predictions and Trends
AI integration into machine learning processes is accelerating, prompting several predictions about future industry trends. Automation of repetitive tasks is expected to increase, freeing machine learning engineers to focus on complex problem-solving and strategy. Gartner predicts that by 2025, 75% of businesses will shift from piloting to operationalizing AI, underscoring a growing demand for advanced technical skills. Engineers with expertise in ethical AI, explainable ML models, and system integration are likely to be in high demand.
Case Studies of Automation in Technology
Numerous instances illustrate AI’s transformative impact on technology sectors through automation. For example, Google’s AutoML automates the creation of machine learning models, reducing the need for manual coding and model selection. This enables engineers to spend more time on innovation and less on routine tasks. In financial services, JPMorgan’s COiN platform leverages AI to perform document review tasks that previously required thousands of hours, significantly improving efficiency and accuracy. In healthcare, IBM Watson uses AI to analyze patient medical records faster than traditional methods, aiding physicians in making informed treatment decisions. These examples highlight how AI-driven automation is reshaping roles and responsibilities within the tech industry.
Preparing for Future Changes
The ongoing evolution of AI is necessitating shifts within the machine learning engineering landscape. Engineers must continuously adapt to remain relevant.
Skills Development for Engineers
Engineers need to focus on acquiring multidisciplinary skills. Core competencies include advanced algorithm development, data analysis, and model optimization. Additionally, proficiency in programming languages such as Python, R, and Julia is crucial. Understanding frameworks like TensorFlow, PyTorch, and Keras provides a competitive edge.
Soft skills also play a vital role in an engineer’s toolkit. Communication abilities are essential for collaborating with cross-functional teams. Problem-solving aptitude and creativity are valuable for developing innovative solutions.
Continuous learning keeps engineers abreast of the latest advancements. Courses, certifications, and attending industry conferences are ways to stay updated. Engaging in projects, participating in hackathons, and contributing to open-source initiatives bolster practical experience.
Companies Adapting to AI Integration
Organizations must evolve to utilize AI’s potential fully. Implementing AI-driven strategies starts with a clear vision. Companies need to invest in research and development, fostering an environment conducive to innovation. Leveraging AI for automation enhances efficiency and drives competitive advantage.
Robust training programs are essential for skill development. Companies can offer workshops, webinars, and on-the-job training tailored to AI and machine learning. Collaborations with academic institutions and tech hubs can provide additional resources and expertise.
Embracing a culture of continuous improvement ensures sustained growth. Measuring the impact of AI initiatives helps refine strategies and maximize results. By staying agile and open to technological advancements, companies can navigate the rapidly changing AI landscape effectively.
Conclusion
AI’s rapid advancements are undeniably transforming the tech landscape. While automation might handle repetitive tasks machine learning engineers remain crucial for tackling complex problems and driving innovation. Their expertise in algorithm development data management and model optimization ensures AI technologies continue to evolve and improve.
As AI continues to grow engineers must adapt by acquiring advanced technical skills and nurturing essential soft skills. Companies should support this growth by investing in research and offering comprehensive training programs. With a collaborative approach AI and machine learning engineers can together shape the future of technology.
Frequently Asked Questions
What is the difference between artificial intelligence and machine learning?
Artificial intelligence (AI) simulates human intelligence, whereas machine learning focuses on training algorithms with data to make predictions.
How do AI and machine learning engineers collaborate?
AI and machine learning engineers work together by creating algorithms, managing data, training models, and evaluating performance to develop and deploy AI solutions.
What are the primary responsibilities of machine learning engineers?
Machine learning engineers develop algorithms, manage datasets, train models, evaluate performance, and deploy solutions to drive innovation and automate processes.
How is AI transforming various sectors?
AI enhances efficiency in sectors like manufacturing (robots), customer service (chatbots), and healthcare (medical diagnostics), performing repetitive tasks with precision.
What are some recent breakthroughs in AI?
Recent AI breakthroughs include deep learning, neural networks, AlphaGo’s victory over humans in Go, the GPT-4 language model, reinforcement learning in robotics, and advanced image recognition in medical diagnostics.
How does automation impact machine learning engineers’ jobs?
Automation handles repetitive tasks, freeing machine learning engineers to focus on complex problem-solving and R&D, requiring advanced technical and multidisciplinary skills.
What skills are essential for machine learning engineers in the AI era?
Machine learning engineers need advanced algorithm development, data analysis, and programming languages like Python, R, and Julia. Soft skills like communication and creativity are also vital.
How should companies adapt to AI integration in the tech industry?
Companies should invest in research and development, provide robust training programs, and foster continuous improvement cultures to leverage AI and maintain competitive advantages.