Machine learning has revolutionized countless industries, making tasks faster and more efficient. From predicting customer behavior to diagnosing diseases, it seems there’s nothing these algorithms can’t tackle. But amidst all the hype, it’s easy to overlook their limitations.
Despite their impressive capabilities, machine learning models aren’t magic. They struggle with understanding context, lack creativity, and often require vast amounts of data to function well. These limitations remind us that while machine learning can augment human abilities, it can’t replace the nuanced understanding and creativity that humans bring to the table.
Understanding Machine Learning’s Limitations
Machine learning excels in many areas but can’t master everything. A clear understanding of its limitations helps manage expectations.
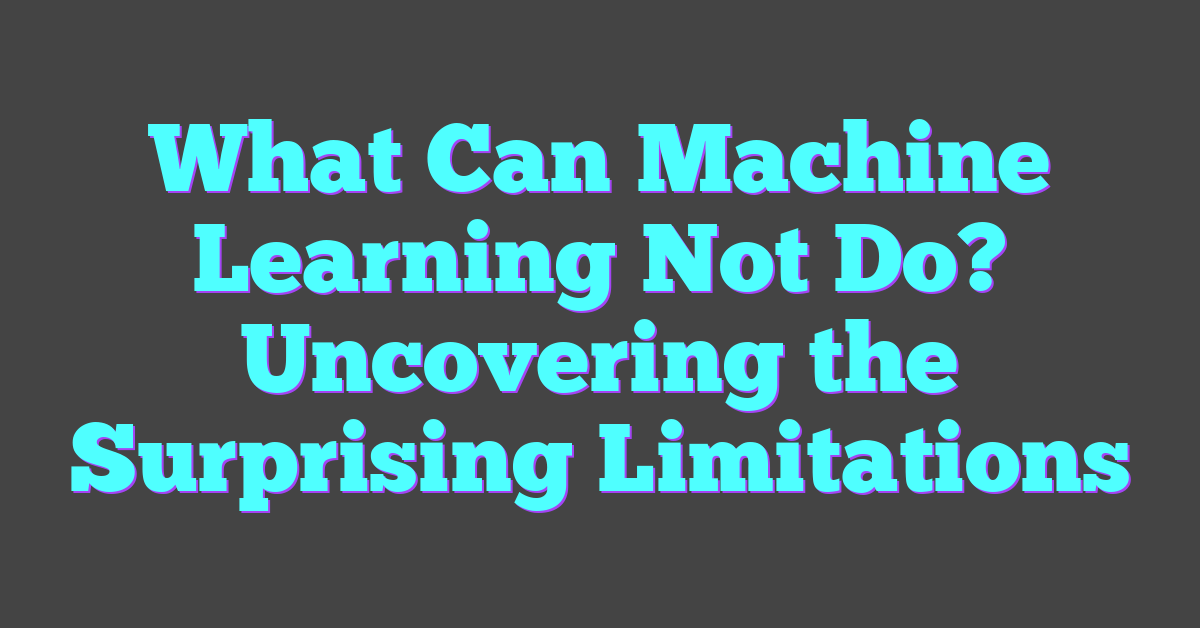
Complex Human Emotions and Decision-Making
Machine learning models can’t understand complex human emotions and decision-making. They analyze data patterns but miss human subtleties. For instance, they can’t recognize sarcasm, irony, or emotions in a conversation. Algorithms also struggle with ethical decisions, as they lack moral reasoning and empathy which are crucial in complex human interactions.
Generalizing From Limited Data
Machine learning struggles to generalize from limited data. Models need vast, diverse datasets to perform well. If the data is insufficient or biased, the algorithms produce unreliable outcomes. For instance, image recognition systems require thousands of labeled images to accurately identify objects. When trained on limited datasets, their accuracy drops significantly, leading to potential errors in real-world applications.
Ethical Concerns in Machine Learning
Machine learning algorithms significantly impact everyday life, but their ethical implications can’t be ignored.
Biases in Training Data
Biases in training data lead to problematic outcomes. If the data used for training reflects societal biases, the algorithm replicates and amplifies these biases. For example, facial recognition systems often show higher error rates for darker-skinned individuals than for lighter-skinned ones. This issue arises from the lack of diversity in the training datasets, which predominantly include lighter-skinned individuals. Consequently, decision-making systems in hiring or law enforcement can unjustly disadvantage certain groups.
Privacy and Surveillance Issues
Privacy and surveillance concerns arise from machine learning applications. For instance, algorithms can analyze large datasets containing personal information, raising the risk of privacy breaches. Social media platforms, for example, collect extensive user data to improve ad targeting. This data collection entails significant privacy risks, as unauthorized access or misuse can compromise user security. Additionally, surveillance systems enhanced by machine learning can lead to invasive monitoring of public and private spaces, potentially infringing on civil liberties.
Technological Boundaries of Machine Learning
Machine learning (ML) offers significant advancements but faces several technological limitations. These boundaries restrict its capabilities, making it crucial to understand where ML falls short.
Dependency on Quality and Quantity of Data
Machine learning heavily relies on the quality and quantity of data. Without extensive, high-quality data, ML algorithms struggle to identify patterns accurately. Poor data can result in biased or incorrect models. For instance, a speech recognition system trained on clear, noise-free recordings may perform poorly on real-world noisy audio. The dependency on data quality also involves the comprehensiveness of the dataset. Models trained on incomplete data often lack the generalization ability for new, unseen situations. This requirement for extensive data often makes the initial setup cost and time-consuming.
Inability to Make Moral Judgments
Machine learning lacks the ability to make moral judgments. Algorithms follow patterns and data without understanding ethical implications. For example, an ML model might optimize for profit in a business scenario without considering ethical practices like fair labor or environmental impact. These algorithms can’t discern right from wrong. They operate based on predefined criteria and cannot adapt to complex human moral standards. This limitation necessitates human oversight to ensure decisions align with societal and ethical norms.
Common Misconceptions About Machine Learning Capabilities
There are several misconceptions surrounding the capabilities of machine learning. This section addresses some of the most prevalent myths and clarifies the actual limitations of this technology.
The Myth of Full Automation
Many believe machine learning can achieve full automation across various tasks. However, it still requires human oversight. While machine learning algorithms perform specific tasks, they depend on predefined parameters and extensive datasets. Additionally, they can’t adapt to unexpected scenarios without human intervention. For instance, autonomous vehicles still need human supervision for safety in unpredictable environments.
AI vs. Human Creativity
Machine learning excels at pattern recognition and data analysis but can’t replicate human creativity. Creativity involves generating novel ideas, emotions, and concepts beyond existing data patterns. For example, AI can assist in composing music or generating art based on existing styles, but it can’t produce genuinely original works that reflect personal experiences or emotions. Humans remain indispensable in fields requiring innovative and imaginative thinking.
Conclusion
While machine learning has undeniably transformed many fields, it’s clear that it has its limitations. It struggles with understanding context, lacks creativity, and requires vast amounts of data to function effectively. Additionally, machine learning can’t grasp complex human emotions or make ethical decisions on its own. Human oversight remains essential to ensure that machine learning systems operate within ethical and societal norms. Though it excels at specific tasks, it can’t fully replicate human creativity or achieve complete automation. Understanding these limitations helps set realistic expectations and guides responsible use of this powerful technology.
Frequently Asked Questions
How has machine learning revolutionized various industries?
Machine learning has enhanced efficiency in numerous industries by improving tasks such as predicting customer behavior and diagnosing diseases. Its ability to analyze large datasets quickly has led to better decision-making and more personalized services.
What are the main limitations of machine learning?
Machine learning struggles with comprehending context, lacks creativity, and requires substantial data for accuracy. It also fails to grasp complex human emotions and ethical considerations, necessitating human oversight for decisions that align with societal norms.
Why does machine learning require large amounts of data?
Machine learning algorithms need vast and diverse datasets to identify accurate patterns and generalize effectively. Limited data can lead to poor performance and unreliable results.
What ethical concerns are associated with machine learning?
Ethical concerns include biases in training data leading to unfair outcomes, privacy issues from analyzing personal information, and surveillance risks from monitoring activities. Ensuring ethical alignment and fairness remains a challenge.
Can machine learning understand human emotions and make ethical decisions?
No, machine learning cannot fully comprehend human emotions or make ethical judgments. It lacks the ability to understand moral implications and requires human oversight to guide ethical decision-making.
Does machine learning operate independently without human intervention?
No, machine learning still requires human oversight. While it excels at specific tasks like pattern recognition, humans are necessary to ensure decisions align with ethical standards and societal norms.
Can machine learning generate creative and original works?
Machine learning falls short in replicating human creativity. It can create variations based on existing data but lacks the ability to produce truly original works that reflect personal experiences and emotions.
Are biases in machine learning training data a significant issue?
Yes, biases in training data can lead to unjust outcomes and perpetuate discrimination. Ensuring diverse and balanced datasets is crucial to mitigating bias and achieving fair results.
How does machine learning impact privacy and surveillance?
Machine learning algorithms can analyze personal information and monitor activities, raising privacy and surveillance concerns. Ensuring responsible use and regulatory oversight is essential to protect individual privacy rights.