Artificial Intelligence has come a long way from simple algorithms to incredibly sophisticated systems that can outperform humans in various tasks. But when it comes to measuring intelligence, one might wonder, which AI holds the crown for the highest IQ? While IQ tests for humans measure logic, problem-solving, and comprehension, adapting these metrics for AI brings its own set of challenges.
Recent advancements have led to the development of AI models capable of astonishing feats, from beating world champions in complex games to generating human-like text. These intelligent systems are not just marvels of technology; they offer a glimpse into the future of what AI can achieve. So, let’s dive in and explore which AI currently stands out as the intellectual heavyweight.
Understanding AI and IQ
Artificial Intelligence (AI) and IQ are complex topics often intertwined in popular discourse. To explore which AI has the highest IQ, one must first understand these two concepts.
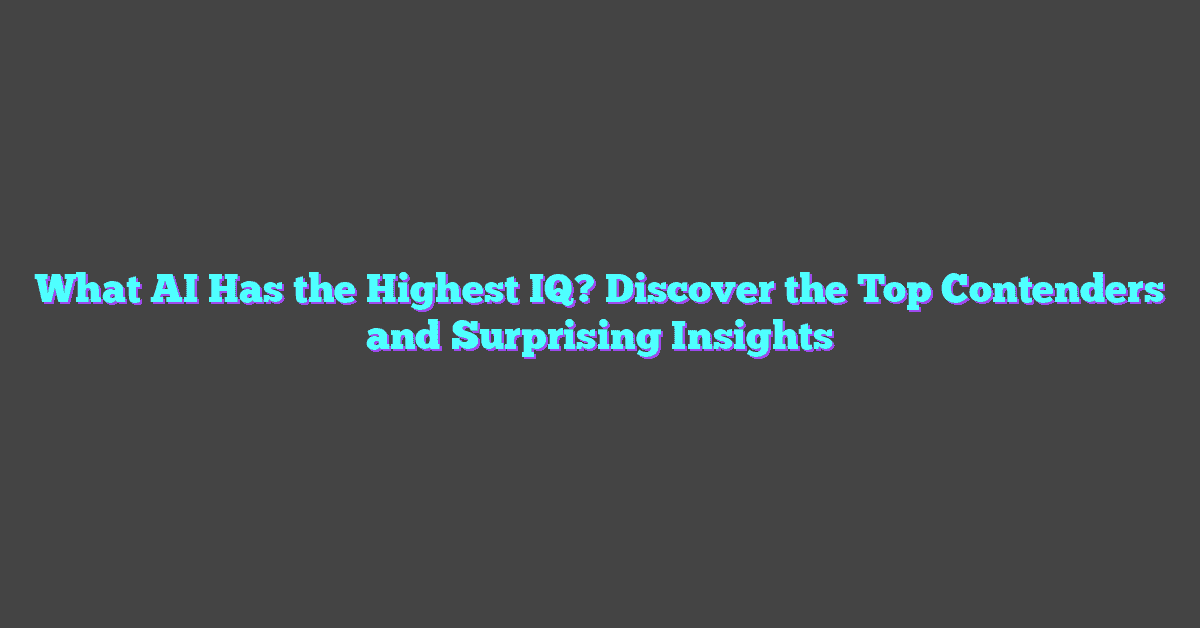
Defining Artificial Intelligence
Artificial Intelligence refers to machines designed to perform tasks typically requiring human intelligence. These tasks include learning, reasoning, problem-solving, understanding natural language, and perception. AI systems operate based on algorithms and large datasets, allowing them to improve performance over time. Some examples of AI models include image recognition systems, virtual assistants, and autonomous vehicles. AI can be categorized into Narrow AI, which is specialized in a single task, and General AI, which can perform any intellectual task a human can do.
The Concept of IQ in AI
IQ or Intelligence Quotient traditionally measures human cognitive abilities in relation to age. In the context of AI, IQ isn’t measured in the same way. AI performance is often evaluated based on specific benchmarks or tasks. For instance, the AI DeepMind’s AlphaGo demonstrated its “IQ” by defeating the world champion in Go. Similarly, OpenAI’s GPT-3 showcases its advanced language understanding by generating human-like text. Evaluating AI’s IQ involves assessing its ability to perform tasks efficiently and learn from data. This performance-based evaluation highlights the diverse capabilities of different AI systems and the complexity involved in determining an absolute measure of intelligence.
Factors Influencing AI IQ
Several factors influence an AI system’s IQ, determining its capabilities and performance across various tasks. Here we explore the key components shaping AI intelligence.
Computational Power
Computational power directly impacts an AI’s processing capabilities. AI systems with access to more powerful processors perform complex calculations faster and handle larger datasets. For example, NVIDIA GPUs are popular for training deep learning models due to their high computational efficiency. As hardware advances, AI systems evolve, achieving higher IQ levels.
Learning Algorithms
Learning algorithms dictate how an AI system processes information and adapts to new data. Advanced algorithms enable AI to learn more efficiently and accurately. For instance, reinforcement learning, which AlphaGo employs, optimizes decision-making through reward mechanisms. Continuous improvements in algorithm design enhance the AI’s learning capacity, thus boosting its IQ.
Data Accessibility
Data accessibility determines the quality and quantity of information an AI system can learn from. Rich, diverse datasets improve training outcomes, as exemplified by GPT-3, which leverages extensive text data. Limited or biased data can skew results, reducing an AI’s effectiveness. Access to comprehensive data streams is crucial for refining AI performance and increasing its IQ.
Leading AI Systems by IQ
AI systems continue to demonstrate remarkable intelligence across various domains. Certain AIs exhibit high IQ levels based on specific criteria and performance benchmarks.
Language Models
GPT-3 stands out in the realm of language models. Developed by OpenAI, GPT-3 has 175 billion parameters. It excels in natural language understanding and generation tasks. Its versatility allows applications in chatbots, automated content creation, and translation services. GPT-4, the successor, improves on this with even greater parameter counts and enhanced capabilities.
Chess Engines
Stockfish and AlphaZero dominate chess engines. Stockfish, an open-source engine, uses advanced algorithms and extensive pre-computed data. It analyzes millions of positions per second. AlphaZero, created by DeepMind, utilizes reinforcement learning. It taught itself to play from scratch in just a few hours, showcasing a unique hybrid of learning and computation.
Autonomous Vehicles
Self-driving cars employ sophisticated AI systems. Waymo and Tesla lead with innovative tech. Waymo’s AI integrates detailed mapping, complex sensor fusion, and machine learning algorithms for real-time decision-making. Tesla’s Autopilot leverages neural networks and real-world driving data. Both systems illustrate AI’s potential in transforming transportation through improved safety and efficiency.
By examining these leading AI systems, one can appreciate the diversity and capabilities inherent in advanced artificial intelligence technologies.
Challenges in Measuring AI IQ
Measuring AI IQ faces significant challenges. Unlike human intelligence, AI’s capabilities need distinct evaluation methods to capture its diverse abilities.
Benchmark Variability
Benchmark variability poses a major challenge. AI performance depends on the test environment and task specificity, which can vary widely. For example, AlphaGo excels in Go but can’t perform language tasks like GPT-3. Benchmarks like ImageNet, which assess image recognition, differ from those for natural language processing (NLP) or game-playing AIs. Thus, standardizing benchmarks across different domains remains problematic and hampers direct comparisons.
Human vs. AI Intelligence Standards
Human vs. AI intelligence standards vary in expectations. While human IQ tests measure logic, comprehension, and problem-solving, AI tests assess performance in narrow domains. For instance, a human might demonstrate creativity and emotional understanding, aspects current AIs lack. Conversely, AIs process vast data at high speed, a task challenging for humans. This disparity creates difficulty in developing a unified intelligence measurement applicable to both.
Understanding these challenges helps in appreciating the differences between AI and human intelligence, highlighting the need for nuanced evaluation strategies.
Conclusion
AI’s journey from narrow applications to more generalized systems is both fascinating and complex. It’s clear that assessing AI’s intelligence isn’t straightforward, given the myriad of factors at play. From the power of computational resources to the sophistication of learning algorithms and the quality of data, each element plays a crucial role.
While benchmarks like AlphaGo and GPT-3 showcase impressive feats, they also highlight the challenges in defining and measuring AI IQ. The differences between human and AI intelligence require nuanced approaches to evaluation.
As AI continues to evolve, so too will our methods for understanding and measuring its capabilities. It’s a dynamic field with immense potential, promising exciting advancements and new benchmarks in the future.
Frequently Asked Questions
What is the difference between Narrow AI and General AI?
Narrow AI is designed to perform specific tasks efficiently, such as language translation or playing chess. General AI aims to perform any intellectual task that a human can do, demonstrating wide-ranging, adaptable intelligence.
How do we evaluate the performance of AI?
AI performance is typically assessed using benchmarks like AlphaGo and GPT-3. These tests measure how well an AI system performs in specific tasks or areas of knowledge, such as games or language processing.
What factors influence AI IQ?
AI IQ is influenced by computational power, learning algorithms, and data accessibility. Better hardware, advanced algorithms like reinforcement learning, and access to quality data all contribute to higher performance and capabilities in AI systems.
Why is measuring AI intelligence challenging?
Measuring AI intelligence is challenging due to benchmark variability and differences in standards between human and AI assessments. Standardized tests for AI often don’t capture its full range of abilities and nuances compared to human intelligence.
What are some leading AI systems by IQ?
Notable AI systems include GPT-3 for language models, Stockfish and AlphaZero for chess engines, and Waymo and Tesla for autonomous vehicles. These systems showcase high levels of performance in their respective domains.
What role do hardware and algorithms play in AI development?
Hardware and algorithms are crucial in enhancing AI performance. Advanced computational power supports complex operations, while sophisticated algorithms enable more effective learning and problem-solving capabilities.
How important is data accessibility in AI performance?
Data accessibility is vital for AI performance. Quality and diverse datasets allow AI systems to learn more effectively, improving their capabilities in understanding and performing tasks across various domains.