Key Takeaways
- AI-Enhanced Procedural Generation: Leveraging artificial intelligence enables the creation of unique and adaptive game levels, enhancing replayability and player engagement.
- Increased Development Efficiency: Automated level creation reduces the time and resources required for manual design, allowing developers to focus on refining game mechanics and storytelling.
- Scalability and Diversity: AI-driven algorithms support expansive and diverse game worlds, offering limitless environments without a proportional increase in development effort.
- Personalized Player Experiences: Machine learning models analyze player behavior to tailor level difficulty and aesthetics, ensuring a customized and enjoyable gaming experience.
- Advanced Generation Techniques: Utilizing neural networks and evolutionary algorithms allows for the creation of intricate and dynamic game environments that adapt in real-time.
- Future Innovations: Ongoing advancements in AI promise more sophisticated procedural generation methods, including real-time adaptation, enhanced personalization, and better integration with emerging technologies like VR and AR.
Procedural generation has transformed how game developers craft immersive worlds. By harnessing artificial intelligence, games now offer endlessly unique levels that adapt to players’ styles and preferences.
This innovative approach not only boosts replayability but also streamlines development. Players embark on fresh adventures with each playthrough, keeping the gaming experience exciting and dynamic.
As AI continues to evolve, the possibilities for procedural generation in game design expand, promising even more engaging and diverse environments for gamers to explore.
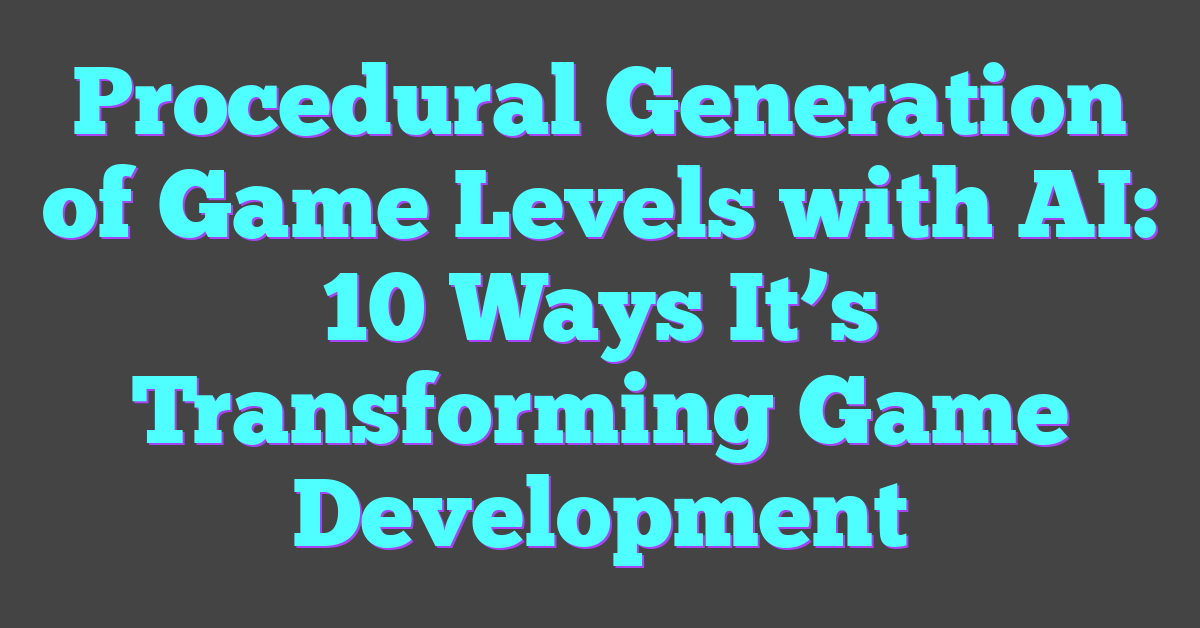
Overview of Procedural Generation
Procedural generation creates game content algorithmically instead of manually. It encompasses environments, levels, items, and more, ensuring diverse and unique experiences each gameplay session.
Key Components
- Algorithms: Define the rules and patterns for content creation.
- Randomness: Introduces variability, making each generated element distinct.
- Constraints: Ensure generated content meets game design requirements.
Types of Procedural Generation
Type | Description |
---|---|
Terrain Generation | Generates landscapes, mountains, valleys, and water bodies. |
Dungeon Generation | Designs maze-like structures with rooms and corridors. |
Item Generation | Produces weapons, armor, and other in-game items. |
Story Generation | Develops plotlines, quests, and narrative elements. |
Benefits
- Replayability: Offers fresh experiences each playthrough.
- Development Efficiency: Reduces time and resources needed for content creation.
- Scalability: Expands game worlds without extensive manual input.
AI Enhancement
Integrating AI into procedural generation refines algorithms, enabling more sophisticated and adaptive content creation. Machine learning models analyze player behavior to tailor game environments, increasing engagement and satisfaction.
Role of AI in Procedural Level Generation
AI transforms procedural level generation by enabling dynamic and adaptive game environments. This integration enhances creativity and streamlines development processes.
Enhancing Creativity
AI-driven procedural generation expands design possibilities through advanced machine learning algorithms. These algorithms analyze extensive datasets to create diverse and intricate game levels that manual design might overlook. For instance, neural networks generate complex dungeon layouts or varied terrain types, ensuring each player experiences a unique environment. Additionally, AI adapts to player behavior, tailoring level complexity and aesthetics to maintain engagement and provide fresh challenges.
Improving Efficiency
Integrating AI into procedural generation significantly reduces development time and resource allocation. Automated level creation allows developers to focus on refining game mechanics and storytelling. Machine learning models rapidly generate and iterate level designs, ensuring a high volume of quality content. Furthermore, AI identifies and rectifies design inconsistencies, maintaining balance and playability across generated levels. This efficiency accelerates the development cycle and enables scalable game worlds that expand seamlessly as the game evolves.
Techniques in AI-Based Procedural Level Generation
AI employs various techniques to generate dynamic and engaging game levels. Two prominent methods are neural networks and evolutionary algorithms.
Neural Networks
Neural networks leverage deep learning to create intricate game environments. By training on extensive datasets, these networks identify patterns and structures within existing levels. For instance, convolutional neural networks (CNNs) can generate complex dungeon layouts by recognizing spatial relationships between rooms and corridors. Recurrent neural networks (RNNs) enable the creation of sequential elements, such as story-driven level progression. Additionally, generative adversarial networks (GANs) produce diverse terrain types by balancing generator and discriminator models, ensuring each level maintains uniqueness and coherence.
Evolutionary Algorithms
Evolutionary algorithms simulate natural selection to evolve game levels over successive generations. These algorithms use processes like mutation, crossover, and selection to optimize level design based on predefined fitness criteria. For example, a genetic algorithm might start with a population of random level layouts, then iteratively refine them by selecting the most playable designs and introducing variations. This approach allows for the automatic discovery of innovative level features and solutions that enhance gameplay. Furthermore, evolutionary strategies can adapt level complexity and difficulty in real-time, providing personalized experiences tailored to individual player skills.
Advantages of Using AI for Level Generation
- Enhanced Creativity: AI algorithms produce diverse level designs, including varied terrains, intricate dungeons, and unique item placements, enriching the gaming experience.
- Increased Efficiency: Automated generation accelerates development by reducing the time and resources needed for manual level creation, allowing teams to focus on refining game mechanics.
- Scalability: AI-driven procedural generation supports expansive game worlds, enabling the creation of large and complex environments without a proportional increase in development effort.
- Personalization: Machine learning models analyze player behavior, tailoring level difficulty and aesthetics to maintain engagement and provide a customized experience.
- Consistency and Quality Control: AI ensures that generated levels adhere to design constraints and balance requirements, minimizing inconsistencies and maintaining high-quality standards.
- Rapid Iteration: Automated processes allow for quick generation and testing of multiple level variations, facilitating faster iteration and optimization based on player feedback.
- Cost-Effectiveness: By minimizing the need for extensive manual design, AI reduces development costs while maintaining or enhancing the quality of game levels.
Challenges and Limitations
Procedural generation with AI introduces several challenges that impact game development:
Quality Control
Ensuring generated levels meet design standards can be difficult. AI might produce elements that lack coherence or balance, requiring extensive testing and refinement to maintain quality.
Computational Resources
AI-driven procedural generation demands significant processing power. High-performance hardware is necessary to handle complex algorithms, potentially increasing development costs and limiting accessibility for smaller studios.
Balancing Randomness and Design
Achieving a balance between randomness and structured design poses a challenge. Levels must remain unpredictable to enhance replayability while adhering to cohesive design principles to ensure enjoyable gameplay.
Player Experience
Maintaining consistency and fairness in gameplay is essential. AI-generated levels must accommodate varying player skills and preferences, preventing frustration from overly difficult or monotonous environments.
Data Dependency
AI models rely on large datasets for training. Acquiring and curating high-quality data is time-consuming and resource-intensive, which can hinder the development process and limit the diversity of generated content.
Integration with Existing Systems
Ensuring compatibility with game engines and existing workflows is critical. Procedural generation tools must seamlessly integrate with other development components to avoid disrupting the production pipeline.
Creativity Constraints
AI may struggle to replicate the nuanced creativity of human designers. While capable of generating diverse content, AI might produce levels that lack the unique artistic vision and storytelling elements crafted by experienced designers.
Debugging Complexity
Identifying and fixing issues in procedurally generated content is more complex than with manually designed levels. The dynamic nature of AI-generated environments makes it challenging to predict and resolve potential problems.
Summary of Challenges
Challenge | Description |
---|---|
Quality Control | Maintaining design standards in generated levels |
Computational Resources | High processing power requirements |
Balancing Randomness | Ensuring unpredictability while adhering to design principles |
Player Experience | Consistency and fairness across diverse player skills |
Data Dependency | Need for large, high-quality datasets |
Integration with Systems | Compatibility with game engines and development workflows |
Creativity Constraints | Limited ability to emulate human creative design |
Debugging Complexity | Difficulty in identifying and fixing generated content issues |
Addressing these challenges involves ongoing research and development to refine AI algorithms, optimize computational efficiency, and enhance integration with game development processes. By tackling these limitations, developers can better harness the potential of AI-driven procedural generation to create engaging and dynamic game levels.
Future Trends in AI Procedural Generation
AI-driven procedural generation continues to evolve, shaping the future of game development with innovative advancements. Emerging trends focus on enhancing personalization, leveraging sophisticated neural networks, and improving real-time generation capabilities.
Enhanced Personalization
AI analyzes player behavior to create tailored game levels. By examining gameplay patterns, AI adjusts difficulty, aesthetics, and layout, ensuring each experience aligns with individual preferences. For example, if a player prefers exploration over combat, the AI generates levels with more intricate pathways and hidden areas.
Advanced Neural Networks
Next-generation neural networks, such as transformers and diffusion models, improve the complexity and diversity of generated content. These models enable the creation of more realistic environments and intricate level designs. For instance, transformers can understand and replicate architectural styles, resulting in cohesive and immersive game worlds.
Real-Time Procedural Generation
Real-time generation allows game levels to adapt dynamically during gameplay. This capability ensures that environments respond to players’ actions and decisions instantaneously. Games like No Man’s Sky demonstrate the potential of real-time procedural generation, offering endless exploration opportunities without repetitive content.
Cross-Genre Adaptability
AI procedural generation adapts to various game genres, from action-adventure to puzzle and strategy games. This flexibility broadens the application of procedural techniques, allowing developers to create unique experiences across different gaming styles. For example, strategy games can utilize AI to generate balanced maps, while puzzle games benefit from diverse level configurations.
Improved AI-Human Collaboration
Tools that facilitate collaboration between AI and human designers streamline the development process. AI handles repetitive tasks, allowing designers to focus on creativity and fine-tuning. This partnership enhances efficiency and ensures that generated content meets high-quality standards. Platforms integrating AI-assisted design tools exemplify this collaborative approach.
Increased Efficiency and Optimization
Advancements in computational efficiency reduce the resources required for procedural generation. Optimized algorithms enable faster generation times and lower energy consumption, making AI-driven processes more sustainable and accessible. Developers can achieve scalable game worlds without compromising performance or quality.
Ethical Considerations and Fairness
Ensuring fairness and avoiding biases in AI-generated content is paramount. Future trends emphasize the development of ethical guidelines and frameworks to govern procedural generation. By addressing potential biases and promoting inclusive design, AI can create more equitable and diverse game environments.
Integration with Virtual and Augmented Reality
AI procedural generation enhances virtual and augmented reality experiences by creating immersive and responsive environments. In VR and AR games, real-time generation ensures that virtual spaces are interactive and engaging, adapting seamlessly to user interactions and movements.
Data-Driven Content Refinement
Continuous data collection and analysis refine procedural generation techniques. By leveraging player feedback and in-game metrics, AI models improve over time, producing more refined and enjoyable content. This iterative approach ensures that procedural generation remains aligned with player expectations and preferences.
Collaborative Open-Source Development
The open-source community contributes to the advancement of AI procedural generation. Shared resources, algorithms, and frameworks accelerate innovation and foster collaboration among developers worldwide. Open-source initiatives promote the exchange of ideas and best practices, driving the field forward collectively.
Future Trend | Description |
---|---|
Enhanced Personalization | Tailoring game levels based on individual player behavior and preferences. |
Advanced Neural Networks | Using transformers and diffusion models for more complex and diverse content generation. |
Real-Time Procedural Generation | Dynamically adapting game environments during gameplay. |
Cross-Genre Adaptability | Applying procedural generation techniques across various game genres. |
Improved AI-Human Collaboration | Streamlining development through AI-assisted design tools. |
Increased Efficiency and Optimization | Reducing computational resources while maintaining high-quality content. |
Ethical Considerations and Fairness | Developing guidelines to ensure unbiased and inclusive AI-generated content. |
Integration with VR and AR | Enhancing immersive experiences through responsive and interactive virtual environments. |
Data-Driven Content Refinement | Continuously improving content based on player feedback and in-game data. |
Collaborative Open-Source Development | Fostering innovation through shared resources and community collaboration. |
Future trends in AI procedural generation promise to revolutionize game development by making it more personalized, efficient, and ethical. As these technologies advance, developers can create richer and more engaging game worlds that adapt to the ever-evolving landscape of player preferences and technological capabilities.
Conclusion
AI-driven procedural generation is transforming the gaming landscape by offering endless possibilities and unique experiences for players. Developers can now craft expansive and varied worlds that keep players engaged and coming back for more. This innovative approach not only boosts creativity but also streamlines the development process, allowing for quicker iterations and improvements.
As technology continues to advance, the collaboration between AI and game designers promises even more immersive and personalized game levels. Embracing these advancements will enable the creation of dynamic environments that evolve with player preferences and gameplay styles. The future of game development is bright, with AI paving the way for richer and more engaging gaming adventures.