In a world where technology evolves at lightning speed, machine learning stands out as a game-changer. It’s not just transforming industries but reshaping how we live, work, and interact. Yet, as with any powerful tool, it brings with it a host of ethical questions. Is it right for machines to make decisions that impact human lives?
From self-driving cars to personalized ads, machine learning algorithms are everywhere, making choices that were once the domain of humans. While the benefits are undeniable, the ethical implications can’t be ignored. Can we trust these systems to be fair and unbiased? And what about privacy concerns? As we dive into the world of machine learning, it’s crucial to explore these ethical dilemmas and consider how they shape our future.
Exploring the Ethical Landscape of Machine Learning
The rapid advancement of machine learning raises critical ethical questions. These concerns impact industries and society at large, requiring careful consideration.
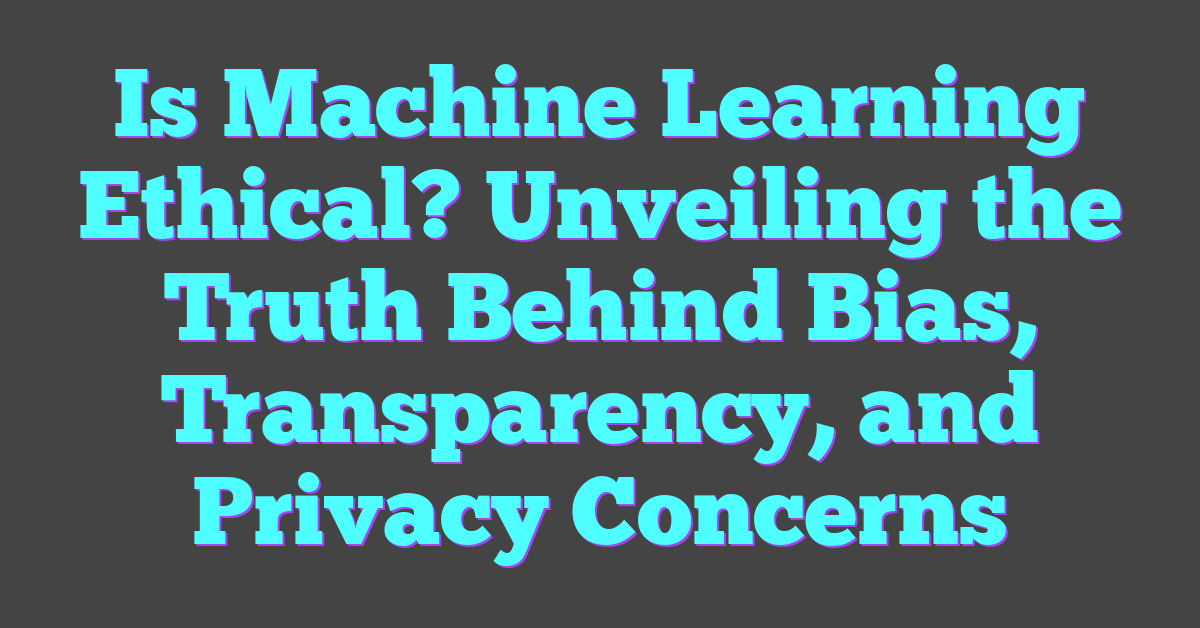
Defining Ethics in AI and Machine Learning
Ethics in AI and machine learning involves principles guiding fair, transparent, and accountable use of algorithms. Developers must ensure systems treat users impartially and respect privacy, embedding these protocols during the design phase. For example, when designing a recommendation system, ethical guidelines ensure all user data is handled securely and recommendations are unbiased and inclusive.
Key Ethical Concerns in Machine Learning
Several ethical concerns arise in the field of machine learning.
- Bias and Fairness: Algorithms can perpetuate or even amplify existing biases present in training data. For instance, facial recognition software often misidentifies individuals from minority groups. Ensuring datasets are diverse and eliminating biased training practices is crucial for fairness.
- Transparency and Explainability: Machine learning models, especially deep learning, can be opaque, making it challenging to understand how decisions are made. Transparency means making the decision-making process clear. Explainable AI (XAI) techniques help users and developers verify outputs effectively. For example, credit scoring systems should provide clear criteria for creditworthiness decisions.
- Privacy Concerns: Machine learning systems often require vast amounts of data, raising privacy issues. Ensuring user data is anonymized and securely stored is vital. Policies like the General Data Protection Regulation (GDPR) enforce strict data handling protocols to safeguard user privacy. In healthcare, for example, patient data requires stringent privacy measures to prevent misuse.
Addressing these key ethical concerns is vital for the responsible deployment of machine learning technologies.
The Bias Problem in Machine Learning Models
Machine learning models carry significant potential for bias, impacting their fairness and effectiveness. Understanding sources of bias and their effects is crucial for developing ethical AI systems.
Sources of Bias in Data
Data Collection: Data collection methods often introduce biases depending on who collects the data, how it’s collected, and under what conditions. For instance, datasets lacking diversity reflect narrower perspectives.
Historical Bias: Historical data inherently contains biases present at the time of its collection. If past decisions were biased, models trained on such data will likely replicate these biases.
Labeling: Human judgment in labeling data leads to subjective biases. Labelers’ perspectives and knowledge affect the labels they assign, creating inconsistencies.
Impacts of Algorithmic Bias
Decision-Making: Bias in algorithms skews decision-making processes, affecting areas like hiring, lending, and law enforcement. For example, biased hiring algorithms may favor specific groups unfairly.
User Experience: Biased algorithms impact user experience on platforms such as social media, search engines, and recommendation systems. Biased recommendations reinforce stereotypes and promote echo chambers.
Legal and Ethical Risks: Biased algorithms expose organizations to legal and ethical risks. Liability issues arise when decisions made by biased models affect individuals’ lives unfairly.
Addressing the bias problem in machine learning involves careful scrutiny of data sources and algorithm design to minimize unfairness and promote ethical use.
Regulatory and Governance Frameworks
Ethical considerations in machine learning necessitate robust regulatory and governance frameworks to guide algorithm development and deployment.
Current Regulations Governing AI
Governments worldwide have started enacting regulations to ensure machine learning systems operate ethically. The European Union’s General Data Protection Regulation (GDPR) sets stringent requirements for data privacy and protection, directly impacting AI and machine learning applications. In the United States, the Algorithmic Accountability Act mandates companies to assess and mitigate the risks associated with automated decision systems.
Some countries have begun creating specific AI-focused regulations. For example, China’s New Generation AI Development Plan aims to position the nation as a global leader in AI while ensuring ethical standards in AI research and applications. These regulations address issues like transparency, accountability, and fairness in AI systems.
The Role of International Bodies
International bodies play a significant role in standardizing and harmonizing AI regulations across borders. Organizations like the United Nations Educational, Scientific and Cultural Organization (UNESCO) have developed guidelines to promote ethical AI development. UNESCO’s Recommendation on the Ethics of Artificial Intelligence outlines principles such as human rights, environmental sustainability, and inclusiveness in AI practices.
The Organisation for Economic Co-operation and Development (OECD) provides another framework with its AI Principles, which advocate for AI systems that are safe, fair, and transparent. These principles guide member countries in formulating policies and regulations that foster trust and promote ethical AI use.
Cross-border collaborations among these organizations are crucial for addressing global challenges in AI ethics. By working together, they can create comprehensive guidelines ensuring that machine learning technologies benefit society while minimizing risks.
Case Studies: Ethics in Action
Exploring real-world applications reveals how ethical concerns in machine learning manifest. Two critical areas are healthcare and surveillance.
Healthcare and Machine Learning Ethics
In healthcare, machine learning drives innovations but raises ethical issues. Bias in training data, for example, can lead to flawed medical recommendations. In 2019, a study published in the journal Science revealed that a widely-used algorithm favored white patients over black patients for special care programs, impacting millions negatively. This discrepancy highlights the necessity for diverse datasets and constant algorithm reviews to ensure fairness.
Additionally, transparency becomes crucial when lives are at stake. Explainable AI models help medical professionals understand and trust machine-generated decisions. Efforts like DARPA’s Explainable Artificial Intelligence (XAI) program aim to make model decision paths clear, enabling better clinical judgment. Without transparency, the black-box nature of some algorithms can erode trust in AI-driven diagnostics.
Surveillance and Privacy Issues
Surveillance technologies harnessing machine learning introduce significant privacy concerns. The deployment of facial recognition systems by law enforcement raises issues of consent and misuse. For instance, an investigation by the New York Times in 2020 showed how Clearview AI’s facial recognition technology scraped billions of images from social media without user consent, raising questions about privacy and data rights.
Moreover, the potential for biased algorithms in surveillance systems to disproportionately target specific groups cannot be ignored. In 2018, MIT Media Lab research found that facial recognition accuracy dramatically decreased for individuals with darker skin tones, leading to wrongful identification and privacy violations. Regulatory measures like the EU’s GDPR aim to mitigate these risks by enforcing strict data protection standards.
Through these case studies, the ethical dimension of machine learning becomes apparent, underscoring the importance of continuous ethical scrutiny and the development of robust frameworks to guide AI applications.
Conclusion
Machine learning’s ethical landscape is complex and multifaceted. As technology advances, it’s crucial to remain vigilant about the ethical challenges it presents. Addressing bias, ensuring fairness, and maintaining transparency are essential steps toward responsible AI development.
Real-world applications in healthcare and surveillance highlight the importance of ethical scrutiny. Diverse datasets and transparent algorithms can help mitigate bias, while robust frameworks are needed to safeguard privacy. Continuous evaluation and adaptation of ethical guidelines will be key to harnessing the full potential of machine learning in a way that benefits society as a whole.
Frequently Asked Questions
What are the main ethical concerns in machine learning?
The main ethical concerns in machine learning include bias, fairness, transparency, explainability, and privacy. These issues can affect how data is processed, decisions are made, and how these decisions impact individuals and communities.
How does bias in machine learning affect healthcare?
Bias in machine learning in healthcare can lead to flawed medical recommendations. This often results from unrepresentative training data, highlighting the need for diverse datasets and regular algorithm reviews to ensure fairness and accuracy in diagnosis and treatment.
Why is transparency important in AI-driven healthcare decisions?
Transparency in AI-driven healthcare decisions is crucial to build trust among patients and medical professionals. Clear understanding and communication of how AI makes decisions can improve the acceptance and reliability of AI applications in medical contexts.
What are the privacy concerns related to surveillance using AI?
Privacy concerns in surveillance using AI arise mainly with facial recognition technology. For instance, companies like Clearview AI have faced criticism for unauthorized scraping of social media images, raising significant privacy issues.
How can ethical issues in machine learning be mitigated?
To mitigate ethical issues in machine learning, it is important to scrutinize data sources, ensure algorithm transparency, conduct rigorous reviews, and implement diverse and inclusive datasets. Continuous ethical scrutiny and robust frameworks are essential to guide AI applications responsibly.