Artificial Intelligence (AI) has made leaps and bounds in recent years, transforming everything from healthcare to finance. But with its growing presence, questions about its accuracy have also surfaced. Can we really trust AI to detect patterns, anomalies, or even diagnose diseases with the same precision as human experts?
While AI’s potential is immense, it’s not without its flaws. Algorithms can be biased, data can be imperfect, and the technology itself is always evolving. As we rely more on AI for critical decisions, understanding its accuracy becomes crucial. Is AI detection truly reliable, or are we placing too much faith in a system that’s still learning? Let’s delve into the intricacies of AI detection and see if it lives up to the hype.
Understanding AI Detection: Overview and Technologies
AI detection identifies patterns, anomalies, and insights using machine learning models and algorithms. This section delves into its basics and the technologies driving it.
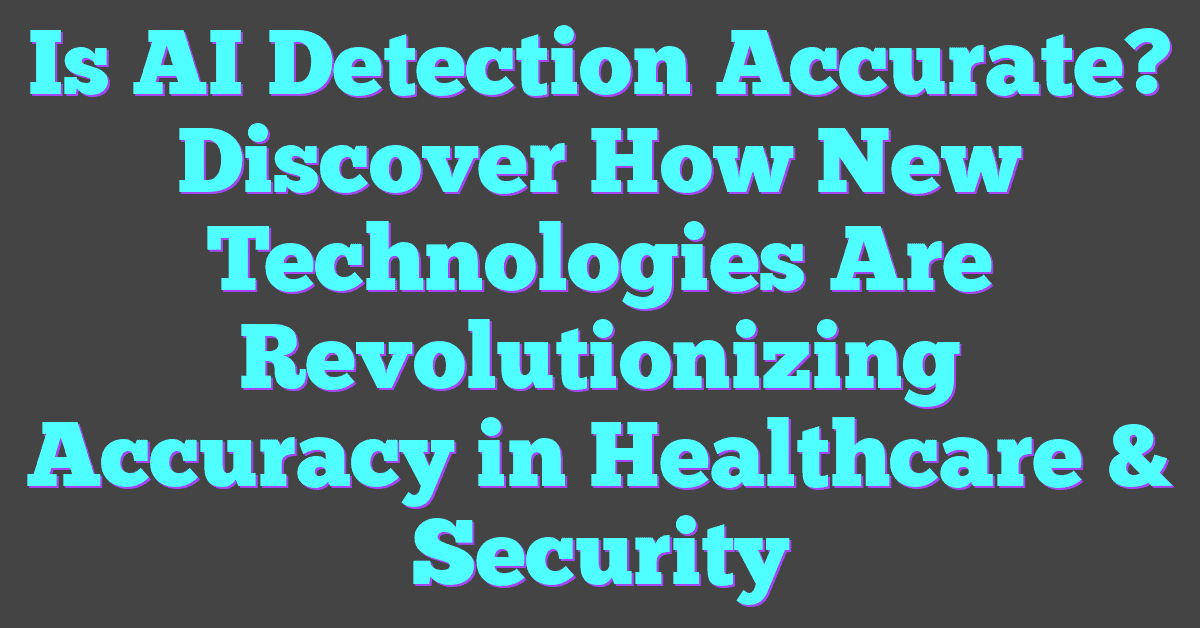
What Is AI Detection?
AI detection refers to using AI models to identify specific patterns or anomalies in data. These models analyze vast datasets to make predictions or detect irregularities. Examples include identifying fraudulent transactions, diagnosing medical conditions, and recognizing speech patterns. Organizations widely leverage it to enhance decision-making processes and increase operational efficiency.
Key Technologies Behind AI Detection
Several technologies power AI detection:
- Machine Learning: AI detection relies heavily on machine learning algorithms which learn from historical data to make predictions. For instance, supervised learning models classify incoming emails as spam based on labeled training data.
- Neural Networks: Complex neural networks mimic human brain structures to analyze intricate data patterns. These networks, including convolutional and recurrent neural networks, are crucial in image and voice recognition.
- Natural Language Processing (NLP): NLP enables AI to understand and interpret human language. Applications like chatbots and sentiment analysis tools use NLP technologies to process and respond to user inputs.
- Computer Vision: This technology allows AI to interpret visual data from the world, crucial in fields like autonomous driving and medical imaging. It involves image recognition, object detection, and facial recognition.
- Big Data Analytics: Handling vast amounts of data efficiently is vital for AI detection. Big data technologies process and analyze large datasets in real time, improving AI detection’s accuracy and speed.
Here’s a brief table summarizing the key technologies:
Technology | Applications |
---|---|
Machine Learning | Spam detection, predictive analytics |
Neural Networks | Image, voice recognition |
Natural Language Processing (NLP) | Chatbots, sentiment analysis |
Computer Vision | Autonomous driving, medical imaging |
Big Data Analytics | Real-time data processing |
These technologies collectively enhance AI detection’s capabilities, making it a powerful tool for various industries.
Evaluating the Accuracy of AI Detection
AI detection plays a crucial role in identifying patterns, anomalies, and generating insights across various industries. Understanding the factors influencing its accuracy is essential for improving its applications.
Factors Influencing AI Detection Accuracy
Several factors affect the accuracy of AI detection systems. High-quality data is a significant factor; algorithms need large, diverse datasets to learn effectively. Data quality plays a vital role because poor data can lead to inaccurate results. Machine learning models depend on these datasets to generalize and perform well under different conditions.
Algorithm complexity is another critical factor. More complex algorithms, such as deep neural networks, generally achieve higher accuracy, but they require more computational resources. Less complex algorithms might be faster but often struggle with accuracy. It’s essential to balance algorithm complexity and available computational power.
Model training and validation processes also impact accuracy. Overfitting occurs when a model performs well on training data but poorly on unseen data. Proper validation techniques, such as cross-validation, help mitigate this issue. Regular updates and continuous learning processes allow models to adapt to new data and improve over time.
Comparisons to Human Performance
AI detection systems exhibit strengths and weaknesses compared to human performance. In medical diagnosis, AI can analyze vast amounts of data quickly, identifying patterns not visible to human eyes. However, it might miss contextual nuances that experienced doctors catch. AI demonstrates high accuracy in repetitive and structured tasks, whereas humans excel in tasks requiring creativity and emotional understanding.
One example is fraud detection. AI systems can monitor and analyze transaction patterns more efficiently than humans. Yet, human experts are essential for interpreting complex cases that require domain-specific knowledge. In speech recognition, AI shows remarkable improvements, though it sometimes struggles with accents and colloquial language, where human understanding prevails.
By evaluating these factors and comparisons, enhancing AI detection’s accuracy becomes more feasible, leading to better outcomes across different applications.
Applications of AI Detection
AI detection plays a crucial role across numerous domains, leveraging advanced technologies to recognize patterns and enhance decision-making. The following sections delve into two significant areas where AI detection has transformative impacts.
AI in Healthcare: Disease and Diagnosis
AI substantially impacts healthcare by improving diagnosis accuracy and disease prediction. Technologies like machine learning and neural networks analyze patient data to identify early symptoms and recommend treatments. For instance, AI systems can review radiology images to detect abnormalities like tumors, often with higher precision than human radiologists. According to a study published in Nature, AI models achieved a diagnostic accuracy of 94.5% in identifying breast cancer from mammograms.
Additionally, AI assists in personalized medicine by analyzing genetic information and predicting patient responses to various treatments. This targeted approach minimizes risks and enhances treatment effectiveness. AI’s ability to sift through massive datasets also supports epidemiology by predicting disease outbreaks and tracking the spread of infections.
AI in Security: Fraud and Threat Detection
AI detection is essential in enhancing security by identifying fraudulent activities and potential threats. Machine learning models analyze transaction patterns to flag anomalies indicative of fraud. For example, financial institutions use AI to monitor credit card transactions, identifying irregular behaviors and preventing unauthorized access. According to a report by McKinsey, AI-based fraud detection systems reduce detection time by up to 70% compared to traditional methods.
In cybersecurity, AI algorithms detect and mitigate threats by continuously monitoring network traffic for suspicious activities. Tools utilizing natural language processing and computer vision identify phishing attempts and malware, safeguarding sensitive information. AI’s predictive capabilities also enhance threat detection, enabling proactive defense strategies against cyberattacks.
AI detection’s role in these areas underscores its potential to improve efficiency and accuracy, making significant strides in both healthcare and security sectors.
Challenges and Limitations of AI Detection
AI detection has limitations that impact its accuracy across various applications. It’s crucial to understand these to maximize the effectiveness of AI-driven solutions.
Technical Limitations and Concerns
AI systems can face technical challenges like data quality and algorithmic biases. Poor data quality affects model performance. In healthcare, an AI model misdiagnoses diseases if trained with incomplete or erroneous patient data. Algorithmic biases occur when training data lacks diversity, causing skewed outputs that can perpetuate misinformation or stereotypes. Computational power is another limitation. Complex models such as neural networks require significant processing resources, limiting deployment in resource-constrained environments.
Ethical and Privacy Issues
AI detection systems raise ethical and privacy concerns. These systems can inadvertently expose sensitive information. For example, in healthcare, AI analyzing patient records might unintentionally reveal personal data. Additionally, bias in data can lead to unfair treatment of specific groups. Ensuring transparency in AI decision-making processes is crucial to maintain trust. Companies must address these ethical issues by incorporating fairness and privacy-preserving mechanisms into AI systems.
Future of AI Detection
AI detection is set to become more sophisticated, transforming industries beyond healthcare and security. Emerging technologies promise groundbreaking changes.
Innovations on the Horizon
Several innovations are reshaping AI detection capabilities. Quantum computing emerges as a key player, offering computational power exponentially greater than classical computers. This advancement accelerates AI training times and enhances predictive accuracy. Edge AI brings real-time processing where data is generated, reducing latency and improving performance in applications like autonomous vehicles. Federated learning allows AI models to train across multiple decentralized devices, enhancing privacy and data security by keeping data local while still improving the model’s accuracy.
Increasing Accuracy and Reliability
The growing accuracy and reliability of AI detection owe much to ongoing research and development. Transfer learning leverages knowledge from pre-trained models to new tasks, improving efficiency and performance. Explainable AI (XAI) strives to make AI decisions transparent, helping users understand and trust AI outputs. Continuous updates and refinements in algorithms, like reinforcement learning, ensure adaptive and resilient AI systems. Collaboration between academia, industry, and government fosters innovation and addresses current limitations, pushing the boundaries of what AI detection can achieve. Transformations in these areas promise to enhance AI’s role in decision-making across varied domains.
Conclusion
AI detection has come a long way and continues to evolve rapidly. While current technologies have shown impressive capabilities, there’s still room for improvement. Emerging innovations like quantum computing and Edge AI are set to revolutionize the field, offering even greater accuracy and reliability.
Collaboration among academia, industry, and government is crucial in driving these advancements. As AI detection technologies become more refined, their applications in healthcare, security, and beyond will only become more impactful.
The future of AI detection looks promising, with ongoing research and development pushing the boundaries of what’s possible. With continuous improvements and new technologies on the horizon, the potential for AI to make a significant difference in various domains is immense.
Frequently Asked Questions
What is AI detection?
AI detection involves using artificial intelligence to identify patterns and anomalies in data, which is crucial for applications like healthcare diagnosis and security.
How does AI improve disease diagnosis?
AI enhances disease diagnosis by analyzing medical data to identify patterns that may go unnoticed by human doctors, increasing accuracy and enabling personalized treatment plans.
What technologies are commonly used in AI detection?
Technologies such as machine learning, neural networks, quantum computing, Edge AI, and Federated learning are commonly used in AI detection to improve accuracy and reliability.
What is the role of machine learning in AI detection?
Machine learning helps AI systems learn from data and improve their detection capabilities over time, making them more accurate and efficient in identifying patterns and anomalies.
How is AI used in security applications?
In security, AI detects anomalies or threats by analyzing vast amounts of data in real-time, which helps in preventing cyber-attacks and enhancing overall safety.
What is Explainable AI (XAI)?
Explainable AI (XAI) refers to AI systems that provide clear and understandable explanations for their decisions, increasing trust and transparency in AI-driven processes.
What is Transfer Learning?
Transfer Learning allows an AI model trained on one task to be adapted for a different but related task, improving performance and reducing the need for large amounts of data.
What are the emerging technologies in AI detection?
Emerging technologies include quantum computing, Edge AI, and Federated learning, which promise to bring groundbreaking improvements in accuracy and reliability of AI detection.
How is collaboration important in AI research and development?
Collaboration across academia, industry, and government drives AI research and development, addressing current limitations and unlocking new potential in various domains.
What challenges does AI detection face?
Challenges include ensuring accuracy, addressing ethical concerns, handling vast amounts of data, and providing explainable results to increase trust and reliability.