Ever wondered how artificial intelligence (AI) gets so smart? From chatbots that understand your queries to recommendation systems that know what you want before you do, AI’s abilities can seem almost magical. But behind the scenes, it’s all about data, algorithms, and a lot of computing power.
AI systems learn and improve through a process called machine learning, where they analyze vast amounts of data to recognize patterns and make decisions. Whether it’s predicting the weather or diagnosing diseases, these intelligent systems rely on complex models and continuous learning to get better over time. Let’s dive into the fascinating world of AI and uncover how these digital brains get their smarts.
Exploring the Concept of “How Do AI Get”
Understanding the process by which artificial intelligence (AI) acquires abilities is crucial. It informs how AI systems are built and how they can be effectively leveraged in various fields.
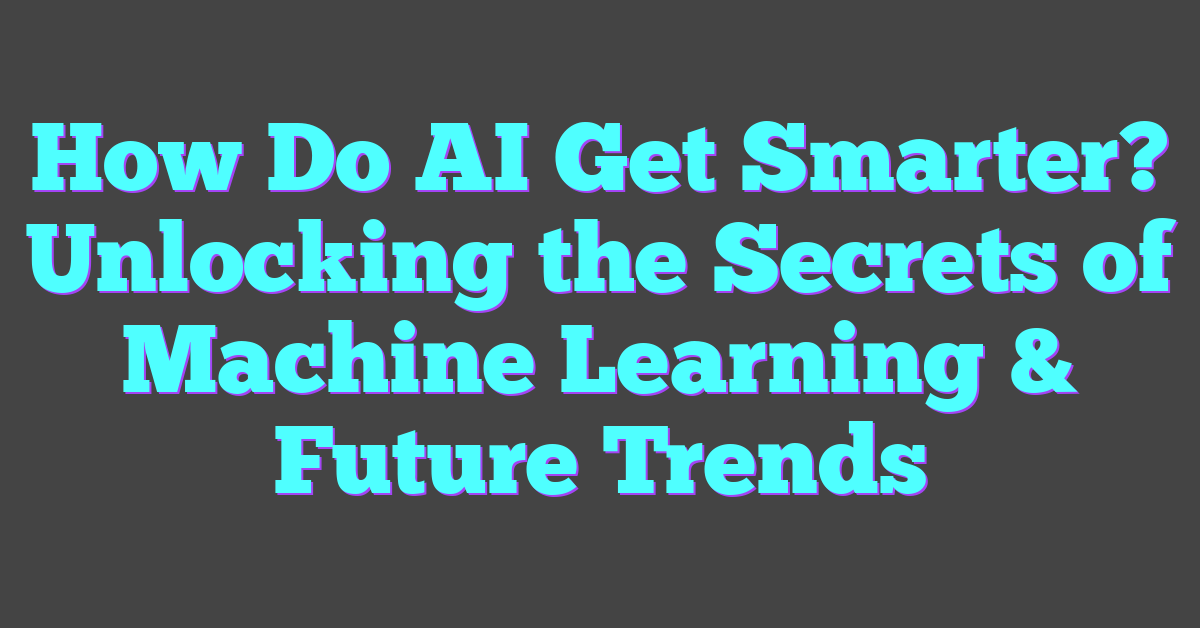
What Does “How Do AI Get” Mean?
The phrase “How Do AI Get” refers to the methods by which AI systems develop their capabilities. This involves machine learning, where algorithms process large datasets to identify patterns and make decisions. By training on diverse data, AI models can perform tasks such as language translation and image recognition. These processes allow AI to improve and adapt to new information, enhancing their utility in different applications.
The Significance of Understanding AI Capabilities
Grasping how AI capabilities are developed is significant for several reasons. It enables better utilization of AI technologies in real-world scenarios. For instance, understanding the training process helps in fine-tuning AI systems for specific tasks, such as natural language processing. Moreover, it aids in identifying potential biases in AI algorithms, ensuring fair and accurate outcomes. By comprehending how AI acquires skills, developers can create more efficient and reliable AI solutions tailored to various industry needs.
Understanding the mechanisms behind AI capabilities also fosters innovation. With a clear grasp of the foundational principles, researchers and developers can push the boundaries of what AI can achieve, leading to advancements in sectors like healthcare and finance. This knowledge is instrumental in harnessing AI’s full potential, paving the way for groundbreaking applications and solutions.
Key Approaches in AI Learning and Development
Machine Learning Models
Machine learning models form the backbone of AI systems, enabling them to learn from data and make predictions. These models include supervised learning, where algorithms are trained using labeled data examples like spam detection, and unsupervised learning, which involves finding patterns in unlabelled data scenarios like customer segmentation.
Reinforcement learning focuses on training models through interactions, using software agents to perform actions and learn from their outcomes, often utilized in gaming and robotics.
Deep Learning and Neural Networks
Deep learning, a subset of machine learning, uses neural networks with multiple layers to process complex data structures. These networks, inspired by the human brain, excel at tasks like image and speech recognition.
Convolutional Neural Networks (CNNs) specialize in analyzing visual data examples, while Recurrent Neural Networks (RNNs) handle sequential data like time-series forecasting. Both types have significantly advanced fields requiring substantial computational power and large datasets.
Challenges and Solutions in AI Development
AI development faces several challenges that experts must address to optimize performance and impact.
Data Collection and Privacy Issues
Collecting data for AI training involves significant challenges. Experts focus on obtaining large, high-quality datasets to improve AI models. While doing so, maintaining privacy is essential. Many regulations, such as GDPR and CCPA, require stringent data handling practices to protect user information. Techniques like data anonymization and federated learning help address privacy concerns. For example, federated learning allows models to learn from data without transferring it to a central server, enhancing privacy.
Algorithm Bias and Ethical Concerns
Algorithm bias poses a significant challenge in AI development. Bias often stems from unrepresentative training data. For instance, if a dataset lacks diversity, the model may produce biased outcomes. Addressing this requires diverse and inclusive datasets. Ethical concerns also arise, particularly in areas like facial recognition and predictive policing. Developers implement fairness-aware algorithms and conduct bias audits to mitigate these issues. Ensuring transparency in AI decision-making processes is another critical solution, helping build trust and accountability in AI systems.
Future Trends in AI Technology
AI technology continues to evolve, introducing groundbreaking advancements and transforming various industries. Optimizing AI autonomy and industry-specific impacts are essential topics within this realm.
Advances in AI Autonomy
Advances in AI autonomy revolutionize machine operations. Autonomous systems, such as self-driving cars and drones, increasingly handle tasks without human intervention. Enhanced algorithms enable these systems to make real-time decisions. For example, self-driving cars navigate through complex environments using sensor data and machine learning models.
Robotic process automation (RPA) also benefits from AI autonomy. Businesses integrate RPA to streamline repetitive tasks, improving efficiency and accuracy. Enhanced with machine learning, RPA systems adapt to new data patterns, resulting in continuous improvement.
Natural language processing (NLP) enhances AI’s autonomy in communication. Chatbots and virtual assistants leverage NLP to understand and respond to user queries, providing real-time support. These capabilities improve user experience by automating customer service and technical support tasks.
Impact of AI Across Different Industries
AI impacts various industries, driving innovation and efficiency. In healthcare, AI aids in diagnosing diseases, developing treatment plans, and personalizing patient care. Algorithms predict patient outcomes using electronic health records (EHRs) and imaging data. For instance, AI systems detect anomalies in medical scans with high accuracy, assisting radiologists.
In finance, AI automates trading strategies, optimizes portfolios, and detects fraudulent activities. Machine learning models analyze market trends and historical data, making real-time trading decisions. AI systems also monitor transactions for irregular patterns, ensuring financial security.
The manufacturing sector sees improvements in production processes through AI. Predictive maintenance, powered by machine learning, reduces downtime by forecasting equipment failures. AI-driven quality control systems inspect products during production, ensuring high standards.
Retail benefits from AI with personalized marketing and inventory management. Recommendation algorithms analyze customer behavior to suggest products, enhancing shopping experiences. Inventory management systems predict demand, optimizing stock levels and reducing wastage.
In logistics, AI improves route optimization and supply chain management. Machine learning models assess variables like traffic and weather to determine efficient delivery routes, reducing costs and delivery times. Automated warehouses use AI for processing orders and managing inventory.
By focusing on specific industry impacts and advances in AI autonomy, it’s clear that AI continues to transform operational landscapes, enhancing efficiency and innovation across multiple domains.
Conclusion
AI continues to evolve, transforming industries and everyday life with remarkable efficiency and innovation. While challenges like data privacy and algorithm bias persist, ongoing efforts in developing diverse datasets, fairness-aware algorithms, and transparent decision-making are paving the way for more trustworthy AI systems. As AI technologies advance, they promise to bring even more autonomy and intelligence to various fields, driving progress and creating new possibilities. Embracing these advancements while addressing ethical concerns will ensure AI’s positive impact on society.
Frequently Asked Questions
What is artificial intelligence (AI)?
AI refers to computer systems that simulate human intelligence to perform tasks like learning, problem-solving, and pattern recognition. It uses data, algorithms, and computing power to improve over time.
How does AI learn?
AI learns through different methods, including supervised learning, unsupervised learning, reinforcement learning, and deep learning, by processing large volumes of data and recognizing patterns.
What is supervised learning?
Supervised learning is a machine learning approach where an AI system is trained on labeled data, meaning the output for each input is known, which helps the system learn to predict outcomes for new data.
What is unsupervised learning?
Unsupervised learning involves training an AI system on data without labeled responses, allowing the system to identify patterns and relationships within the data on its own.
What is reinforcement learning?
Reinforcement learning is a type of machine learning where an AI system learns by receiving rewards or penalties for actions it performs, optimizing its behavior to maximize rewards over time.
What are neural networks?
Neural networks are AI models inspired by the human brain, consisting of layers of interconnected nodes (neurons) that process and analyze data, used in deep learning for complex pattern recognition tasks.
What are the main challenges in AI development?
The main challenges in AI development include data collection and quality, privacy concerns, algorithm bias, and addressing ethical issues to ensure fair, transparent, and accountable AI systems.
How is privacy protected in AI systems?
Privacy is protected using techniques like data anonymization, where identifying information is removed, and federated learning, where data is processed locally on devices without sharing raw data.
What are fairness-aware algorithms?
Fairness-aware algorithms are designed to minimize bias and discrimination in AI systems by incorporating fairness constraints and performing regular bias audits to ensure equitable outcomes.
How is transparency ensured in AI decision-making?
Transparency in AI decision-making is ensured by making AI systems’ processes and decision criteria clear and understandable, often through explainable AI techniques that provide insights into how decisions are made.
What are some future trends in AI technology?
Future trends in AI include increased autonomy for systems like self-driving cars, drones, and robotic process automation, as well as broader applications across various industries to enhance efficiency and innovation.
How does AI impact different industries?
AI impacts industries like healthcare, finance, manufacturing, retail, and logistics by improving operational efficiency, driving innovation, and providing capabilities like predictive analytics, automated processes, and enhanced customer experiences.