In the rapidly evolving world of technology, machine learning stands out as a field full of promise and potential. Many aspiring data scientists and tech enthusiasts often wonder if a PhD is essential to break into this exciting domain. While advanced degrees can open doors, the landscape of machine learning offers diverse pathways to success.
With the rise of online courses, bootcamps, and hands-on projects, individuals now have more opportunities than ever to build a career in machine learning without a traditional academic route. This article explores whether a PhD is truly necessary and highlights alternative ways to thrive in this dynamic field.
Understanding Machine Learning and Education Requirements
Artificial intelligence, specifically machine learning, has become pivotal in today’s tech-driven world. For individuals passionate about AI and content creation, exploring this field without necessarily having a PhD is a viable option.
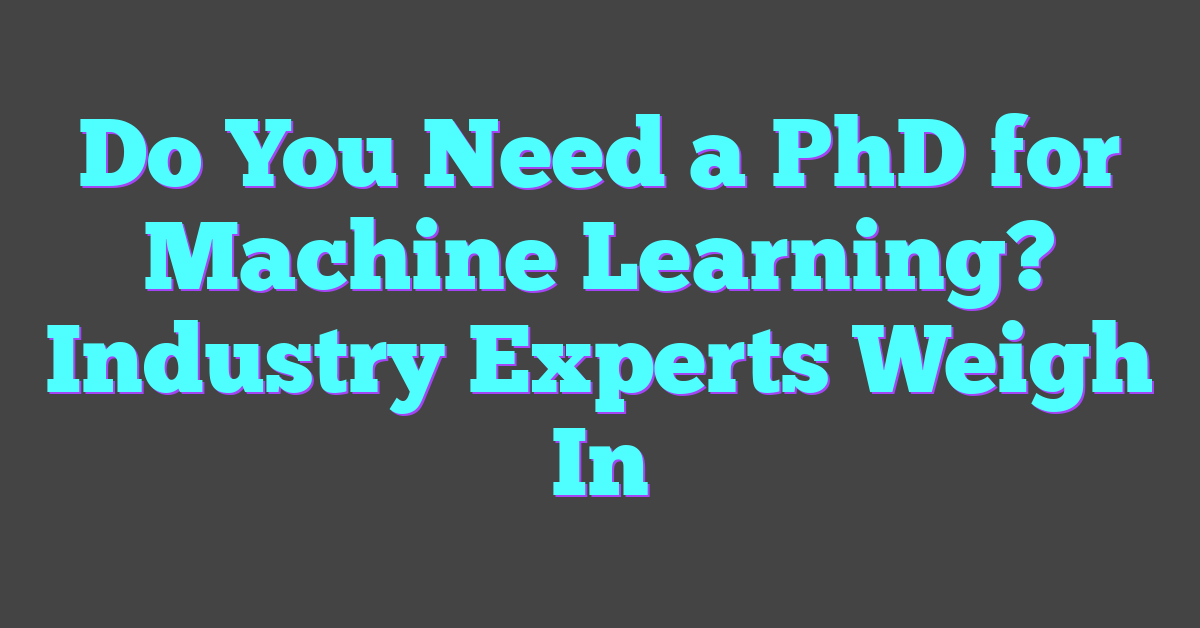
What Is Machine Learning?
Machine learning (ML) is a subset of artificial intelligence focusing on developing algorithms that can learn and make decisions from data. Unlike traditional programming, where rules are explicitly programmed, ML algorithms identify patterns to perform tasks. Fascinating applications include personalized recommendations, autonomous driving, and image recognition.
- Traditional Academic Path
A bachelor’s degree in computer science, mathematics, or a related field provides a strong foundation in algorithms and data structures. For those looking into research or advanced roles, a master’s degree or PhD can enhance expertise and open doors in academia and specialized industry positions. - Online Courses and Certifications
Platforms like Coursera, edX, and Udacity offer courses covering ML fundamentals to advanced topics. Completing these courses and obtaining certifications can build solid ML knowledge and practical skills. For instance, Andrew Ng’s ML course on Coursera is highly regarded. - Bootcamps
Intensive ML bootcamps, such as those offered by General Assembly or Springboard, provide hands-on experience with real-world projects. These programs often include mentorship, which can be invaluable for career switchers or those looking to gain practical skills quickly. - Self-Learning and Projects
Many ML enthusiasts dive into self-learning using free resources, textbooks, and open-source projects. Participating in Kaggle competitions or contributing to GitHub repositories showcases skills and builds real-world experience.
Comprised of these diverse educational paths, aspiring ML practitioners can tailor their learning journey based on personal preferences, career goals, and available resources.
The Role of a PhD in Machine Learning
Pursuing a PhD in Machine Learning can provide in-depth knowledge and extensive research experience, benefiting those aiming for specialized roles. However, it’s not the only pathway to success in this field.
Advantages of Having a PhD
A PhD offers several advantages in the machine learning domain. For instance, candidates with a PhD often gain access to advanced research opportunities that allow them to explore novel algorithms and techniques. Many universities, such as Stanford and MIT, provide cutting-edge resources and mentorship for this purpose.
PhD holders also have a higher likelihood of securing academic positions and research scientist roles in prestigious institutions and corporate labs. According to the Bureau of Labor Statistics, the median salary for computer and information research scientists is over $122,000 annually, a common destination for those with a PhD.
PhD programs emphasize critical problem-solving skills and advanced theoretical knowledge, which are highly beneficial for designing complex machine learning models. For example, breakthroughs in neural networks and natural language processing often come from academic research led by PhD scholars.
What Can You Achieve Without a PhD?
Individuals can achieve significant milestones in machine learning without a PhD. Many professionals enter the field with a strong foundation in computer science, which can be built through a bachelor’s or master’s degree. Industry roles like data scientist, machine learning engineer, and AI specialist are accessible with this level of education.
Online courses and certifications from giants like Coursera and edX equip learners with practical skills and project experience. Additionally, bootcamps like those from General Assembly and Springboard offer intensive, hands-on training that mirrors industry demands.
Self-learning is another viable route. Numerous free resources and open-source projects provide practical experience in areas like deep learning and reinforcement learning. Community forums and GitHub repositories often serve as excellent platforms for networking and collaboration.
Many successful AI professionals have risen to prominence through alternative learning paths. Andrew Ng, co-founder of Coursera, advocates for continuous self-improvement and believes practical experience can make a substantial impact.
Overall, while a PhD offers distinct advantages, it’s not the sole avenue to a rewarding career in machine learning.
Alternative Educational Paths for Machine Learning Careers
Individuals can pursue various educational paths to enter machine learning careers. Exploring these alternatives can provide flexibility and tailored learning experiences.
Bootcamps and Online Courses
Bootcamps offer intensive, short-term training programs designed to equip participants with practical skills for machine learning. These programs typically last 3-6 months and cover fundamental topics like Python, data processing, and model building. Notable bootcamps include Flatiron School, Springboard, and General Assembly. Many graduates secure roles such as data scientist or machine learning engineer.
Online courses, available through platforms like Coursera and edX, offer flexibility and affordability. Learners can choose from a wide range of topics, from introductory courses to advanced algorithms. Institutions like Stanford and MIT provide these courses, often taught by leading experts. Certificates from such courses can enhance resumes, building credibility in the field.
Master’s Degrees and Their Impact
A master’s degree in machine learning or related fields like Data Science provides a structured curriculum that covers both theoretical and practical aspects of the domain. Programs typically last 1-2 years and include coursework in subjects like statistics, machine learning algorithms, and deep learning.
Earning a master’s degree can significantly boost career prospects. Graduates often move into higher-paying roles, such as senior data scientist or machine learning engineer. Universities like Carnegie Mellon, Stanford, and the University of California, Berkeley offer renowned programs in these fields.
Practical projects and internship opportunities during a master’s program enable students to gain real-world experience, making them more competitive in the job market. Many companies prefer candidates with a master’s degree for roles involving complex problem-solving and in-depth analysis.
By considering these educational paths—bootcamps, online courses, and master’s degrees—individuals can find routes that align with their career goals and personal circumstances in the field of machine learning.
Insights From Industry Experts
Industry experts share valuable perspectives on pursuing a PhD in machine learning. These insights help individuals make informed decisions about their educational paths.
Case Studies of Successful Professionals in Machine Learning
• Andrew Ng: Andrew Ng, co-founder of Coursera and former head of Baidu AI Group, holds a PhD in computer science from UC Berkeley. His academic background provided a strong foundation for his career in AI research and higher-level roles.
• Geoffrey Hinton: Renowned as the “Godfather of Deep Learning,” Geoffrey Hinton earned his PhD from the University of Edinburgh. His contributions to neural networks and deep learning have significantly impacted the AI field.
• Sebastian Thrun: Sebastian Thrun, CEO of Udacity and founder of Google X, received his PhD from the University of Bonn. He leveraged his doctoral education to lead transformative AI projects, including self-driving cars.
How Companies View PhDs in Hiring Decisions
• Research Roles: Companies like Google, Facebook, and IBM prioritize candidates with PhDs for research positions. These advanced roles often involve pioneering new AI methodologies and enhancing existing algorithms.
• Leadership Positions: PhDs are often considered for leadership roles within AI teams. An advanced degree showcases expertise and the ability to spearhead complex projects.
• Applied AI Roles: Although a PhD can be advantageous, companies also value practical experience. Many firms, including startups and mid-sized tech companies, hire candidates with strong project portfolios and relevant work experience.
• Alternative Qualifications: Some organizations, such as NVIDIA and Microsoft, recognize alternative educational paths. These firms consider candidates with master’s degrees, industry certifications, or demonstrated self-taught skills in addition to traditional academic credentials.
Conclusion
Whether or not you need a PhD for a career in machine learning depends on your goals and the path you choose. A PhD can open doors to research and leadership roles but it’s not the only way to succeed. Many professionals thrive through alternative routes like online courses bootcamps and self-learning. Companies value both academic credentials and practical experience so it’s essential to find the right balance for your career aspirations. Ultimately the journey in machine learning is diverse and flexible allowing for various paths to success.
Frequently Asked Questions
Do I need a PhD to work in machine learning?
No, a PhD is not necessary to work in machine learning. While a PhD can be beneficial for research roles, many professionals succeed through alternative routes like online courses, bootcamps, and self-learning.
What are the benefits of pursuing a PhD in machine learning?
A PhD in machine learning can open doors to advanced research positions, leadership roles, and academic careers. It also provides a deep understanding of theoretical concepts and cutting-edge techniques.
Can I become a machine learning engineer without a PhD?
Yes, many successful machine learning engineers do not have a PhD. Practical experience, strong programming skills, and completing relevant projects can be enough to start a career in this field.
How do online courses and bootcamps compare to traditional academic routes in machine learning?
Online courses and bootcamps offer flexible, targeted learning paths that can quickly equip you with practical skills. Traditional academic routes provide a more comprehensive theoretical foundation and research opportunities.
What do companies look for when hiring machine learning professionals?
Companies value a mix of practical experience, academic credentials, and problem-solving skills. While a PhD can be important for research roles, strong programming abilities, relevant project experience, and alternative qualifications are also highly regarded.
Are there successful machine learning professionals without PhDs?
Yes, many successful professionals like Andrew Ng, Geoffrey Hinton, and Sebastian Thrun have varied educational backgrounds. Their success demonstrates that practical experience and expertise can outweigh formal qualifications.
How important is research experience in the field of machine learning?
Research experience is crucial for roles focused on innovation and developing new algorithms. It is highly valued for obtaining leadership positions in academic and high-tech research settings.
Can self-learning be effective for a career in machine learning?
Absolutely. Many industry experts have utilized self-learning to gain the necessary skills for a successful career in machine learning. Dedication, reputable resources, and practical application are key components.