Artificial Intelligence (AI) has undoubtedly revolutionized various aspects of our lives, from smart assistants to self-driving cars. However, like any technology, it’s not without its challenges. While AI promises efficiency and innovation, it also brings a set of common problems that can’t be ignored.
From biases in algorithms to concerns about data privacy, AI presents a complex landscape that requires careful navigation. Understanding these issues is crucial for anyone looking to leverage AI responsibly and effectively. Let’s delve into some of the most prevalent problems associated with AI and explore how they impact our world.
Understanding Common Problems with AI
While AI has significantly improved various aspects of modern life, it also introduces several common problems that must be navigated.
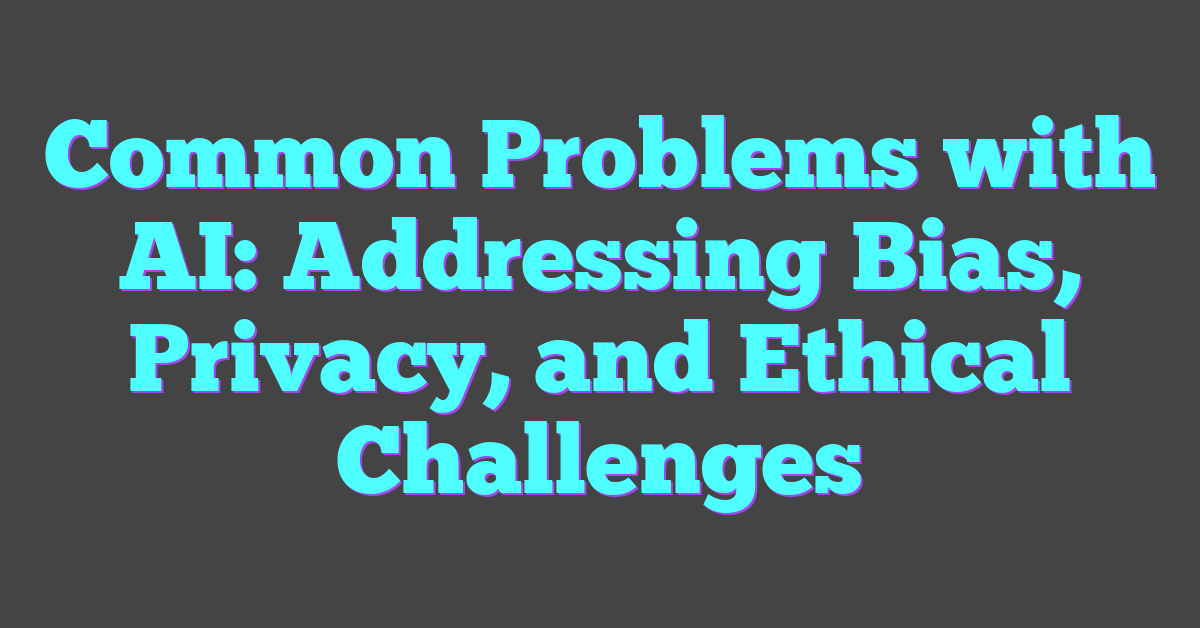
Ethical Concerns and Bias
AI systems often display biases based on the data used to train them. Training on skewed datasets can lead algorithms to make prejudiced decisions, adversely affecting certain groups. For example, facial recognition systems have shown higher error rates for people with darker skin tones. Ethical concerns also arise regarding the use of surveillance data, which can infringe on privacy and civil liberties.
Addressing these issues requires diverse, representative datasets and ethical guidelines. Initiatives like the AI Ethics Guidelines from the European Commission can provide frameworks to ensure fairness and mitigate biases in AI systems.
Lack of Transparency and Explainability
AI models, especially deep learning ones, often operate as “black boxes,” making it difficult for users to understand how decisions are made. This lack of transparency hampers trust and can lead to resistance in adopting AI technologies.
Explainable AI (XAI) aims to resolve this by making AI’s decision-making processes more interpretable. Techniques like LIME (Local Interpretable Model-agnostic Explanations) help provide insights into model decisions, fostering greater accountability and trust.
By emphasizing transparency and explainability, users can better understand AI systems, leading to more responsible and effective deployment.
Technical Challenges in AI Development
Technical challenges frequently arise in AI development, representing significant hurdles to its effective implementation.
Data Quality and Quantity Issues
AI systems rely on vast amounts of high-quality data to learn and make accurate predictions. Poor quality data, including incomplete or noisy datasets, can lead to erroneous outputs. Insufficient data quantity limits the AI model’s ability to generalize, reducing its effectiveness. For instance, biased datasets can skew the performance of facial recognition systems, often leading to misidentification. Regular access to clean, comprehensive datasets ensures AI models perform optimally.
Integration and Scalability Difficulties
Integrating AI solutions into existing systems presents substantial obstacles. Compatibility issues between AI frameworks and legacy systems can impede seamless integration. Scalability is another critical challenge. As the size and complexity of data grow, scaling AI models to handle increased loads becomes essential. Efficiently scaling AI operations requires robust infrastructure and continuous optimization. Failure to address these difficulties may result in limited AI adoption and suboptimal performance in real-world settings.
Socio-Economic Impacts of AI
Artificial Intelligence (AI) significantly influences socio-economic structures worldwide. This section addresses common socio-economic challenges associated with AI.
Job Displacement Concerns
Job displacement due to AI automation presents a significant socio-economic challenge. Industries such as manufacturing, retail, and customer service increasingly adopt AI-driven technologies (e.g., robots, chatbots). Consequently, workers in these sectors face potential job losses. According to a 2020 study by the World Economic Forum, AI could displace 85 million jobs by 2025. However, it may also create 97 million new roles, mainly in AI and other emerging tech sectors. The challenge remains in reskilling and upskilling the workforce to adapt to these changes. Governments, companies, and educational institutions must collaborate on training programs to aid this transition.
Impact on Social Interaction
AI impacts social interaction, primarily through communication tools like chatbots, virtual assistants, and social media algorithms. These tools personalize user experiences but can also create echo chambers by reinforcing existing beliefs. For instance, social media platforms (e.g., Facebook, Twitter) use AI algorithms that curate content based on user preferences, potentially limiting exposure to diverse viewpoints. Additionally, reliance on virtual assistants (e.g., Amazon Alexa, Google Assistant) can reduce human-to-human interaction, impacting social skills and community engagement. Balancing AI benefits with the need for genuine social interaction is essential for societal well-being.
Advances in Addressing AI Challenges
Addressing AI challenges is crucial for its responsible adoption. Recent advancements focus on improving explainability and mitigating biases.
Improvement in AI Explainability
Enhancements in AI explainability increase transparency and trust. Explainable AI (XAI) models use techniques like LIME and SHAP to clarify decision-making processes. For instance, LIME breaks down model predictions to show feature contributions, while SHAP values attribute the impact of each feature on an output. These methods assist users in understanding and verifying AI decisions, boosting confidence in AI applications.
Efforts to Mitigate Bias
Mitigating bias in AI involves innovative approaches. Diverse training datasets and fairness-aware algorithms help address biases. Techniques like reweighting data samples ensure balanced representation of different groups, while adversarial training identifies and minimizes biases during model development. By incorporating these methods, developers can create fairer AI systems, promoting ethical and equitable AI use across sectors.
Conclusion
AI’s potential to revolutionize society is undeniable, but it’s not without its hurdles. Addressing biases, ensuring data privacy, and enhancing transparency are crucial steps toward responsible AI use. By focusing on explainability and fairness, we can build trust and create more equitable systems. As AI continues to evolve, balancing technological advancements with ethical considerations will be key to maximizing its benefits while safeguarding societal well-being.
Frequently Asked Questions
What are the main challenges of Artificial Intelligence (AI) mentioned in the article?
The article highlights challenges such as biases, data privacy concerns, and lack of transparency in AI models. It emphasizes the need for responsible AI use to mitigate these issues.
Why is Explainable AI (XAI) important?
Explainable AI (XAI) is crucial because it enhances the interpretability and trust in AI systems. XAI helps clarify decision-making processes, making AI more transparent and reliable.
What techniques are being used to improve AI explainability?
Techniques like LIME (Local Interpretable Model-agnostic Explanations) and SHAP (SHapley Additive exPlanations) are used to improve the explainability of AI models. These techniques make AI decisions more understandable.
How can biases in AI be mitigated?
Biases in AI can be mitigated by using diverse training datasets and fairness-aware algorithms. These practices aim to create fairer AI systems, ensuring ethical and equitable use across different sectors.
What ethical issues are associated with AI?
Ethical issues in AI include biases in facial recognition, data privacy concerns, and the need for transparency. The article stresses the importance of adhering to AI Ethics Guidelines to address these concerns.
What are the socio-economic impacts of AI mentioned in the article?
The socio-economic impacts of AI include potential job displacement and changes in social interaction. The article calls for a balance between AI benefits and genuine human interaction to ensure societal well-being.
What is the role of diverse training datasets in AI development?
Diverse training datasets help in reducing biases within AI systems. Using varied data sources ensures that AI models are fairer and more representative of different populations.
How do fairness-aware algorithms contribute to AI?
Fairness-aware algorithms are designed to reduce biases in AI decision-making processes. They help ensure that AI applications operate in an equitable manner, promoting fairness across various sectors.
Why is transparency important in AI models?
Transparency in AI models is essential for building trust and accountability. Transparent AI allows users to understand how decisions are made, fostering greater confidence in AI technologies.
What initiatives are mentioned to promote ethical AI use?
The article mentions initiatives like the AI Ethics Guidelines, which provide a framework for ethical AI use. These guidelines are essential for developing and deploying AI responsibly.