Artificial Intelligence (AI) often feels synonymous with machine learning, but are they really inseparable? Many people assume you can’t have one without the other, yet there’s more to AI than meets the eye. While machine learning has certainly driven many recent advancements, it’s just one approach among many in the AI toolkit.
From rule-based systems to expert systems, AI existed long before machine learning became the buzzword it is today. These traditional methods rely on predefined rules and logic rather than data-driven learning. So, can you have AI without machine learning? Absolutely, and understanding this distinction opens up a fascinating world of possibilities.
Understanding AI Fundamentals
AI, or Artificial Intelligence, involves creating systems capable of performing tasks that typically require human intelligence. These tasks include reasoning, learning, and problem-solving.
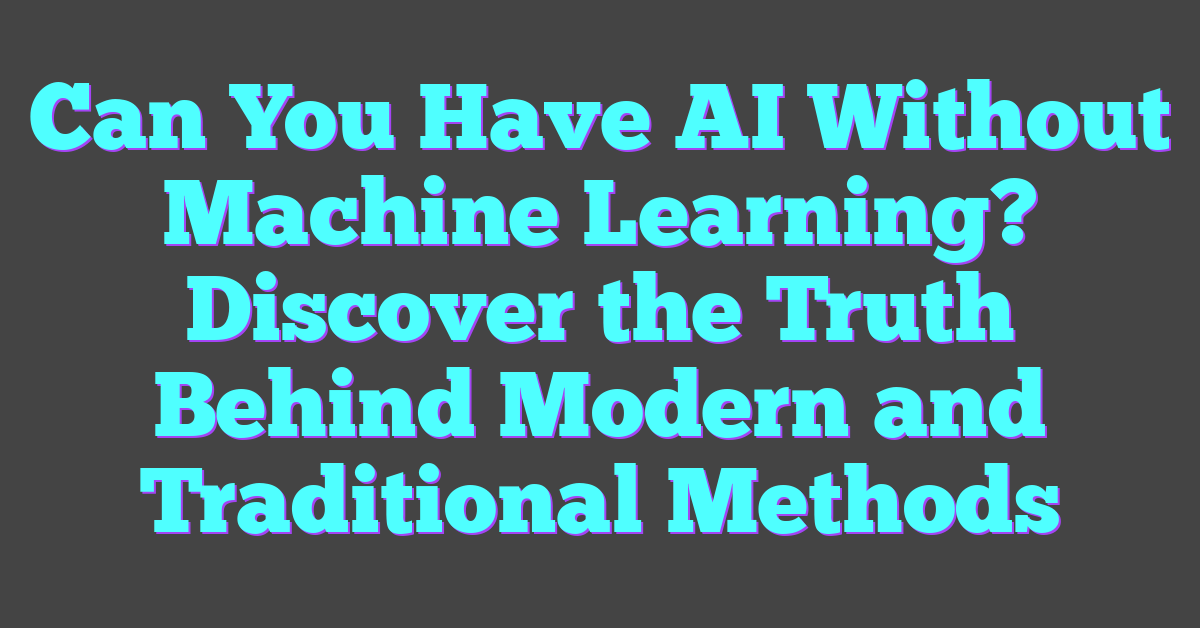
What Is AI?
AI encompasses a broad range of technologies and approaches aimed at replicating human cognitive functions. It includes natural language processing (NLP), computer vision, and robotics, among others. These technologies allow AI to interact with the real world, understand human language, and make autonomous decisions. One popular example is the use of chatbots in customer service, which can handle a variety of queries through NLP.
- Rule-Based Systems: These systems rely on hand-crafted rules to make decisions. They’re effective in controlled environments where rules are clearly defined. An example is an expert system used for medical diagnosis based on predefined symptom rules.
- Expert Systems: Developed in the 1970s, expert systems use a knowledge base of human expertise to make decisions. These systems can perform specific tasks at the level of a human expert. One example is MYCIN, an early expert system for identifying bacterial infections and recommending antibiotics.
- Symbolic AI: This involves manipulating symbols to represent knowledge. It leverages logic and algorithms to solve problems. An example is a chess-playing program that uses predefined strategies and algorithms to compete against human players.
While modern AI often uses machine learning, these traditional approaches highlight the versatility and depth of AI beyond data-driven models.
The Role of Machine Learning in Modern AI
Machine learning is crucial to many contemporary AI applications, enabling systems to learn from data and improve over time.
How Machine Learning Powers AI
Machine learning algorithms allow AI systems to process vast amounts of data to identify patterns and make decisions. Unlike traditional rule-based systems, which require explicit programming, machine learning systems use statistical methods to infer rules from data. This capability makes machine learning essential for complex tasks like image recognition and natural language processing.
Examples of AI Using Machine Learning
- Image Recognition: Systems like Google’s DeepDream generate artistic visuals. This AI identifies patterns and objects within images by training on millions of labeled examples.
- Natural Language Processing (NLP): Tools such as GPT-3, developed by OpenAI, can generate human-like text. This AI understands context and semantics after training on extensive datasets.
- Recommendation Systems: Platforms like Netflix and Amazon use machine learning to recommend content. These AI systems analyze user behavior and preferences to provide personalized suggestions.
- Autonomous Vehicles: Companies like Tesla employ AI in self-driving cars. These systems interpret sensor data to navigate and make driving decisions in real-time.
Integrating Machine Learning and Traditional AI
While machine learning enhances AI’s capabilities, integrating it with traditional AI methods yields robust systems. Expert systems, for instance, use machine learning to refine knowledge bases and improve decision-making processes. This hybrid approach leverages the strengths of both methodologies, confirming a more holistic AI solution.
Exploring AI without Machine Learning
Exploring AI sans machine learning reveals how diverse and versatile artificial intelligence can be. This section delves into two primary methods: Rule-Based Systems and Evolutionary Algorithms.
Rule-Based Systems
Rule-based systems, also known as expert systems, predate contemporary machine learning. These systems operate based on a set of predefined rules, often represented as “if-then” statements. For instance:
- Medical Diagnosis: Expert systems assist in diagnosing diseases by following a set of rules derived from medical knowledge.
- Customer Support: Chatbots provide responses to standard queries using predefined replies.
These systems rely heavily on extensive knowledge databases curated by experts. While they lack the ability to learn from new data, they offer consistent and predictable outputs.
Evolutionary Algorithms
Evolutionary algorithms use principles from biological evolution, such as mutation and selection, to solve optimization problems. These algorithms are instrumental in scenarios where conventional methods fall short. Examples include:
- Optimization Tasks: Used in engineering to find optimal solutions for design problems.
- Game Development: Helps in creating AI opponents that adapt strategies over generations.
Unlike machine learning, evolutionary algorithms iteratively improve solutions without explicitly “learning” from a dataset. They explore numerous possible solutions, gradually honing in on the most effective one through simulated evolution processes.
By understanding these alternative AI methods, the capabilities and breadth of artificial intelligence become clearer. AI systems can exist and function effectively even without the integration of machine learning.
Challenges and Limitations
Exploring AI without machine learning reveals numerous challenges and limitations impacting effectiveness and efficiency. While alternative AI methods exist, their technical limitations and practical implications can constrain AI capabilities.
Technical Limitations Without Machine Learning
AI methods excluding machine learning face significant technical hurdles. Rule-based systems rely heavily on predefined rules, making them inflexible and unable to adapt to new data. Unlike machine-learning models, which learn from data, rule-based systems need constant updates for every new scenario.
Evolutionary algorithms offer optimization for specific problems but lack generalizability. These algorithms perform well in narrow domains, yet struggle with tasks requiring extensive knowledge and adaptability. Additionally, they involve extensive computation and may not find the optimal solution within a feasible timeframe.
Lack of data-driven learning in AI systems without machine learning reduces their ability to handle complex tasks. While these systems may perform specific functions well, they often falter when faced with dynamic and unpredictable environments. By not leveraging data, they’re unable to improve performance over time in varied contexts.
Practical Impact on AI Capabilities
Omitting machine learning from AI curtails its practical applications. For instance, rule-based expert systems in medical diagnostics must be manually updated. As new medical knowledge and treatments emerge, the system becomes outdated quickly, impeding its utility.
In customer support, rule-based chatbots can handle standard queries but can’t understand nuanced customer interactions. This limitation leads to increased reliance on human agents for complex queries, reducing efficiency and scalability.
Evolutionary algorithms, while useful in engineering optimization, cannot handle tasks such as natural language processing or image recognition with the same accuracy and efficiency as machine learning models. This restricts their use in applications demanding real-time data analysis and decision-making.
Finally, the absence of machine learning restricts the development of AI in predicting trends and making recommendations. Systems without machine learning can’t offer personalized experiences based on user data, limiting their effectiveness in industries like e-commerce and entertainment.
While AI without machine learning provides some solutions, the limitations in technical flexibility and practical applications hinder the evolution of robust, adaptive AI systems capable of meeting diverse and dynamic demands.
Conclusion
AI’s potential is vast and varied, but without machine learning, its adaptability and effectiveness are significantly limited. Traditional AI methods can handle specific tasks, yet they struggle with dynamic environments and personalization. Machine learning enables AI to learn from data, evolving and improving over time, making it indispensable for modern applications. While alternative AI methods have their place, integrating machine learning creates more robust and versatile systems. The future of AI lies in its ability to adapt and offer real-time solutions, a feat largely driven by the power of machine learning.
Frequently Asked Questions
What is Artificial Intelligence (AI)?
Artificial Intelligence (AI) refers to the simulation of human intelligence in machines programmed to think and learn like humans. This encompasses technologies such as natural language processing, robotics, and machine learning.
How does traditional AI differ from modern AI?
Traditional AI relies on predefined rules and algorithms, while modern AI incorporates machine learning, enabling systems to learn from data and improve over time, making them smarter and more efficient.
What role does machine learning play in AI?
Machine learning is crucial in modern AI for allowing systems to adapt and improve from experience without explicit programming. It underpins many contemporary AI applications, enhancing their capability to process vast amounts of data and generate insights.
Can you provide examples of AI applications using machine learning?
Yes, examples include image recognition systems that identify objects in photos, and recommendation systems that suggest products or content based on user behavior and preferences.
What are the challenges of AI without machine learning?
AI systems without machine learning face significant technical hurdles, such as limited adaptability and real-time data analysis. They struggle with dynamic environments and cannot offer personalized experiences, limiting their practical applications.
Why are rule-based systems and evolutionary algorithms limited?
Rule-based systems and evolutionary algorithms lack the flexibility required to handle dynamic environments effectively. They are limited by their predefined rules and inability to learn from new data, impacting their use in complex, real-world scenarios.
How does the absence of machine learning affect AI’s effectiveness?
Without machine learning, AI systems are less adaptable and struggle with evolving tasks. They cannot improve over time or provide personalized responses, which limits their usability in applications like medical diagnosis and customer support.