Key Takeaways
- Revolutionizing Design Concepts: AI enables architects to generate innovative and diverse design solutions beyond traditional methods.
- Enhanced Creativity and Efficiency: Leveraging generative design and machine learning algorithms accelerates the creation of multiple design variations quickly.
- Improved Accuracy and Sustainability: Data analytics optimize building performance and resource usage, promoting sustainable and functional designs.
- Advanced Visualization Tools: AI-powered 3D modeling and virtual reality enhance client communication and facilitate better design validation.
- Streamlined Workflows: Automation software reduces repetitive tasks, allowing architects to focus on creative and personalized aspects of their projects.
- Future Innovation Trends: Integration with BIM, smart urban planning, and AI-driven material innovation are shaping the next generation of architectural design.
AI is reshaping the architectural landscape by revolutionizing concept generation. Architects now leverage AI to brainstorm and visualize innovative designs that were once unimaginable. This powerful collaboration between human creativity and machine intelligence is unlocking new potentials in the field.
By harnessing AI, designers can explore a multitude of design variations quickly, identifying unique solutions that enhance both aesthetics and functionality. It’s not just about speeding up the process; AI inspires fresh perspectives, enabling architects to push the boundaries of traditional design. As this technology continues to evolve, the future of architectural concept generation looks more exciting than ever.
Overview of AI in Architectural Design
AI transforms architectural design by automating concept generation processes. Machine learning algorithms analyze vast datasets, identifying patterns and trends that inform innovative designs. Generative design tools create multiple design alternatives based on specified parameters, enhancing creativity and efficiency. AI-powered visualization software enables real-time 3D modeling and virtual reality experiences, facilitating better client communication and design validation. Additionally, data-driven insights optimize building performance, ensuring sustainability and functionality. These technologies streamline workflows, reduce errors, and accelerate the design cycle, enabling architects to focus on refining and personalizing their projects.
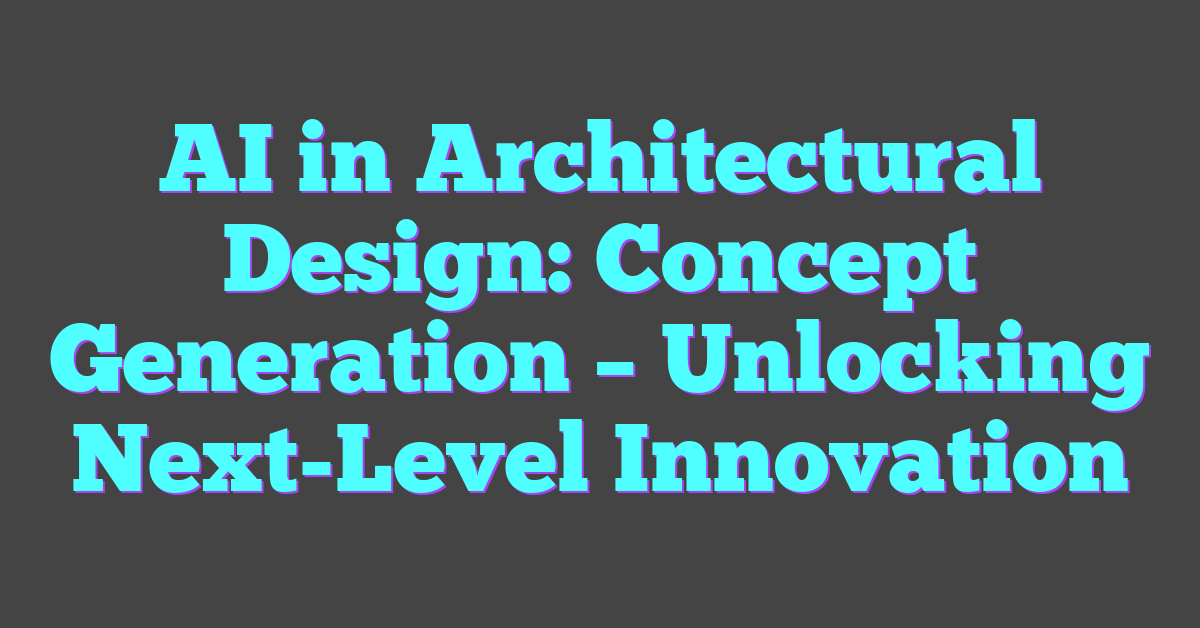
Key Components of AI in Architectural Design
- Machine Learning Models: Analyze historical and real-time data to predict design outcomes.
- Generative Design Algorithms: Produce diverse design options based on user-defined criteria.
- Visualization Tools: Offer immersive 3D models and virtual reality simulations for enhanced client presentations.
- Data Analytics Platforms: Provide insights into building performance, energy efficiency, and material usage.
- Automation Software: Streamline repetitive tasks, allowing architects to concentrate on creative aspects.
Benefits of AI Integration
Benefit | Description |
---|---|
Enhanced Creativity | Generates unique design solutions beyond traditional methods. |
Increased Efficiency | Accelerates the design process by automating routine tasks. |
Improved Accuracy | Reduces human errors through precise data analysis and simulations. |
Sustainable Design | Optimizes resource usage and promotes eco-friendly building practices. |
Better Collaboration | Facilitates seamless communication between stakeholders through advanced visualization tools. |
AI’s integration into architectural design fosters a synergy between human ingenuity and machine intelligence. This collaboration not only elevates the quality of architectural projects but also opens new avenues for innovation and sustainability in the built environment.
AI-Driven Concept Generation Tools
AI-driven tools streamline the concept generation phase in architectural design. These tools enhance creativity and efficiency by leveraging advanced technologies.
Machine Learning Algorithms
Machine learning algorithms analyze extensive architectural data to identify design patterns. Neural networks predict optimal layouts based on historical project outcomes. Decision trees classify design elements, facilitating rapid concept development. Clustering algorithms group similar design features, enabling architects to explore diverse styles. These algorithms ensure data-driven decision-making, enhancing design accuracy and innovation.
Generative Design Software
Generative design software generates multiple design alternatives based on predefined parameters. Tools like Autodesk’s Generative Design, Rhino’s Grasshopper, and Bentley’s GenerativeComponents lead the market. These platforms allow architects to input constraints such as space requirements, material types, and environmental factors. The software produces optimized designs that balance aesthetics, functionality, and sustainability.
Software | Features | Common Uses |
---|---|---|
Autodesk Generative Design | Parameter-based design, optimization algorithms | High-rise buildings, urban planning |
Rhino Grasshopper | Visual scripting, customization | Complex geometries, bespoke structures |
Bentley GenerativeComponents | Parametric modeling, integration with BIM | Infrastructure projects, large-scale developments |
Generative design software accelerates the exploration of innovative solutions, enabling architects to select the best-fit concepts efficiently.
Benefits of AI in Concept Generation
AI revolutionizes architectural concept generation by introducing advanced tools that enhance creativity and streamline workflows. These benefits lead to more innovative and efficient design processes.
Enhanced Creativity
AI-driven tools expand creative possibilities by generating diverse design options. Architects can explore numerous configurations that might be overlooked manually. Key enhancements include:
- Generative Design Algorithms: Produce multiple design alternatives based on specific parameters, enabling unique solutions tailored to project needs.
- Predictive Analytics: Identify trends and patterns from large datasets, inspiring novel architectural concepts.
- Real-Time Visualization: Facilitate immediate feedback on design iterations, allowing for rapid experimentation and refinement.
- Collaborative Platforms: Integrate inputs from various stakeholders, fostering a multidisciplinary approach to creativity.
These capabilities empower architects to push the boundaries of traditional design, resulting in more innovative and aesthetically pleasing structures.
Increased Efficiency
AI optimizes the architectural design process by automating repetitive tasks and accelerating decision-making. Efficiency gains include:
- Automated Layout Optimization: Quickly generates optimal spatial arrangements, reducing the time spent on manual adjustments.
- Data-Driven Insights: Leverages large datasets to inform design choices, ensuring decisions are based on accurate and relevant information.
- Resource Management: Predicts material usage and project timelines, enhancing planning and reducing waste.
- Error Reduction: Minimizes design inconsistencies and mistakes through precise algorithmic calculations.
These efficiency improvements lead to faster project completion times, cost savings, and higher-quality designs.
Challenges and Considerations
Implementing AI in architectural concept generation presents several challenges and important considerations for the industry.
Data Requirements
AI systems rely on extensive, high-quality data to generate accurate design concepts. Acquiring diverse datasets that cover various architectural styles, materials, and environmental conditions is essential. Data preprocessing steps—such as cleaning, normalization, and annotation—ensure machine learning models perform effectively. Additionally, maintaining data security and addressing privacy concerns are crucial when handling sensitive architectural information. Architects must invest in robust data infrastructure to support these requirements and ensure data integrity and accessibility.
Integration with Traditional Methods
Blending AI with conventional architectural practices requires strategic alignment with existing workflows. Architects must balance machine-generated designs with human creativity to preserve the artistic elements of architecture. Training personnel to proficiently use AI tools and software is vital for effective integration. Furthermore, ensuring compatibility between AI systems and current design platforms facilitates a seamless transition. Aligning AI-driven processes with traditional project management methods enhances overall productivity and minimizes disruptions in the design process.
Future Trends in AI for Architectural Design
AI continues to shape the future of architectural design through several emerging trends. These trends enhance creativity, efficiency, and sustainability in the field.
Integration of AI and Building Information Modeling (BIM)
Combining AI with BIM systems improves data management and project coordination. AI algorithms analyze BIM data to predict project outcomes and optimize workflows. This integration enables real-time updates and enhances collaboration among stakeholders.
Advanced Generative Design Techniques
Generative design evolves with AI advancements, allowing for more complex and innovative architectural solutions. Machine learning models generate multiple design iterations based on specific criteria such as material usage, structural integrity, and environmental impact. Tools like Autodesk’s Generative Design and Rhino’s Grasshopper leverage these techniques to produce highly optimized designs.
Enhanced Sustainability through AI
AI-driven analytics promote sustainable architecture by optimizing energy consumption and reducing waste. Predictive models assess building performance under various environmental conditions, guiding designers to make eco-friendly decisions. Sustainable materials and energy-efficient systems are increasingly integrated into AI-generated designs, supporting green building standards.
AI-Powered Virtual and Augmented Reality
Virtual Reality (VR) and Augmented Reality (AR) technologies, enhanced by AI, offer immersive design experiences. AI algorithms personalize virtual environments based on user preferences and design specifications. These technologies facilitate better visualization, client presentations, and design validation, improving overall project outcomes.
Automated Construction Processes
AI automates construction tasks, streamlining the transition from design to build. Robotics and AI-driven machinery handle repetitive and precise tasks such as bricklaying, welding, and assembly. This automation reduces construction time, minimizes errors, and lowers labor costs, contributing to more efficient project execution.
Smart Urban Planning
AI supports smart urban planning by analyzing large datasets to optimize city layouts and infrastructure. Predictive analytics assess factors like population growth, traffic patterns, and environmental impact. This data-driven approach enables the creation of sustainable and resilient urban environments, addressing the challenges of modern city development.
Personalized Architectural Solutions
AI enables the creation of personalized architectural designs tailored to individual preferences and needs. Machine learning algorithms analyze user data to generate customized layouts, aesthetics, and functionalities. This trend enhances user satisfaction and ensures that buildings meet specific requirements and lifestyles.
Enhanced Collaboration Platforms
AI enhances collaboration platforms by facilitating seamless communication and data sharing among project teams. Intelligent systems manage project timelines, track progress, and predict potential issues. These platforms ensure that all team members are aligned, improving project efficiency and reducing the likelihood of delays.
Integration of IoT and AI
The Internet of Things (IoT) integrates with AI to create smart buildings equipped with sensors and automated systems. AI analyzes data from IoT devices to optimize lighting, heating, security, and maintenance. This integration results in buildings that are more responsive to occupants’ needs and environmental changes.
Continuous Learning and Adaptation
AI systems in architectural design continuously learn from new data and project outcomes. Machine learning models adapt to evolving design trends and user feedback, improving their predictive capabilities and design suggestions over time. This ongoing learning process ensures that AI tools remain relevant and effective in a dynamic architectural landscape.
Utilization of AI in Historic Preservation
AI assists in the preservation and restoration of historic buildings by analyzing structural integrity and suggesting appropriate conservation methods. Machine learning models identify potential risks and recommend maintenance strategies, ensuring the longevity of cultural heritage sites.
Expansion of AI-Driven Material Innovation
AI accelerates material innovation by discovering and testing new construction materials. Machine learning algorithms analyze material properties and performance, identifying alternatives that offer better durability, sustainability, and cost-effectiveness. This trend supports the development of advanced building materials tailored to specific project requirements.
Predictive Maintenance and Lifecycle Management
AI enables predictive maintenance by forecasting when building systems require servicing or replacement. Machine learning models analyze data from building operations to predict failures and optimize maintenance schedules. This proactive approach reduces downtime, extends the lifespan of building components, and lowers maintenance costs.
AI in Regulatory Compliance
AI streamlines regulatory compliance by automating the analysis of building codes and standards. Intelligent systems ensure that designs adhere to local regulations, zoning laws, and safety standards. This automation minimizes the risk of non-compliance and accelerates the approval process for architectural projects.
Collaborative AI Design Assistants
AI design assistants collaborate with architects by providing real-time suggestions and enhancements during the design process. These assistants leverage machine learning to understand design preferences and offer alternatives that align with the project’s goals. This collaboration fosters a more efficient and creative design environment.
Data-Driven Aesthetic Enhancements
AI analyzes aesthetic trends and user preferences to inform design choices, ensuring that buildings are visually appealing and culturally relevant. Machine learning models identify patterns in successful architectural styles and apply these insights to generate aesthetically pleasing designs that resonate with target audiences.
AI-Enhanced Accessibility Features
AI improves building accessibility by designing spaces that cater to diverse needs. Intelligent systems analyze accessibility requirements and integrate features such as automated ramps, adaptive lighting, and smart navigation aids. These enhancements ensure that buildings are inclusive and user-friendly for all occupants.
Conclusion
Future trends in AI for architectural design emphasize the integration of advanced technologies to enhance creativity, efficiency, and sustainability. These developments position AI as a pivotal force in shaping the next generation of architectural innovation.
Conclusion
AI is revolutionizing architectural concept generation by merging human creativity with machine precision. This collaboration fosters innovative and sustainable designs that push the boundaries of traditional architecture.
Looking ahead AI promises to further enhance the design process empowering architects to create even more inspiring and efficient structures that shape the skylines of tomorrow